
Selin Nur Barlak
-
|
|
|
May 17, 2022
May 17, 2024
May 17, 2022
May 17, 2022
Marketing and Analytics
Marketing and Analytics
Marketing and Analytics
5
5
5
5
min reading
min reading
min reading
min reading
Data
Data
Data
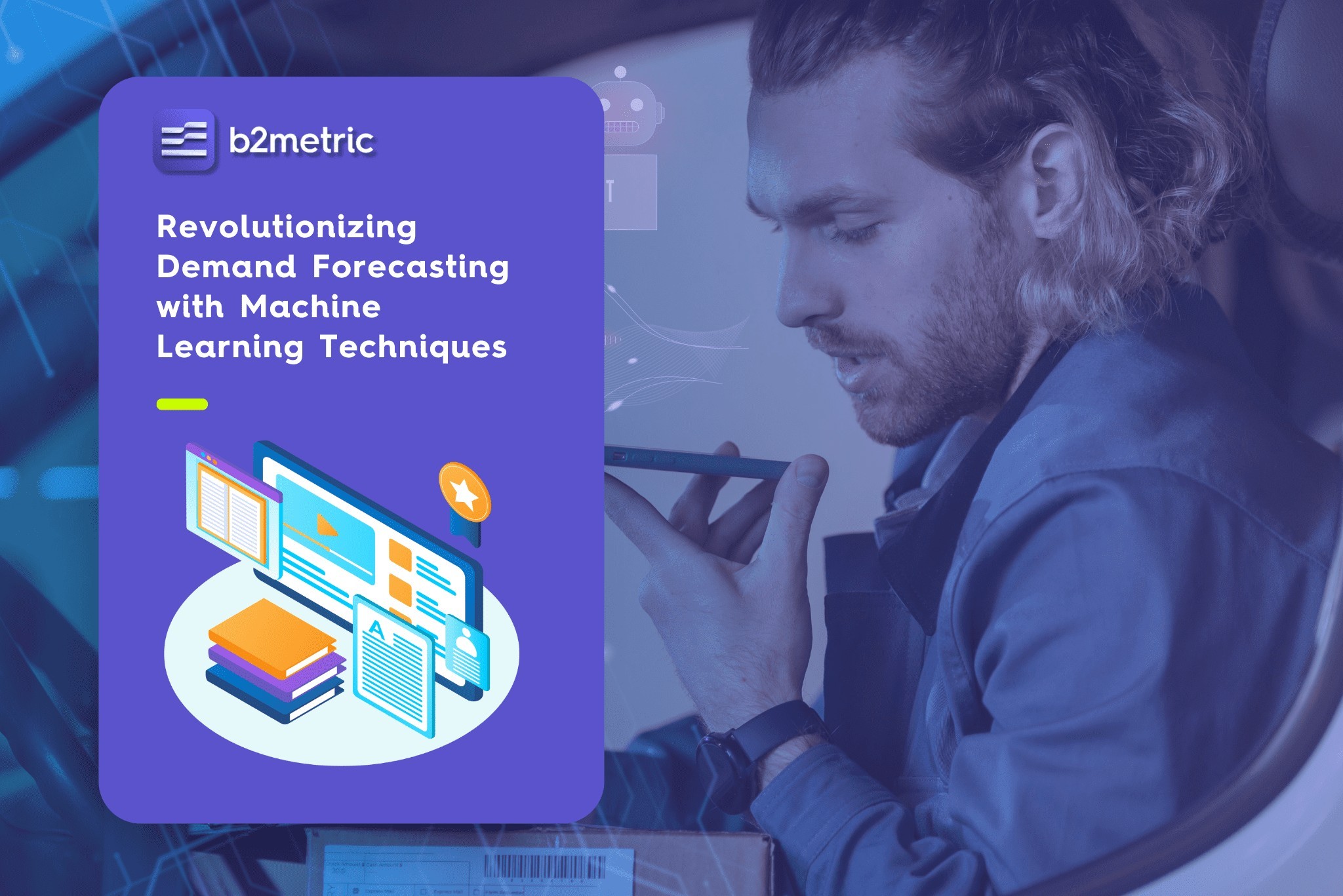
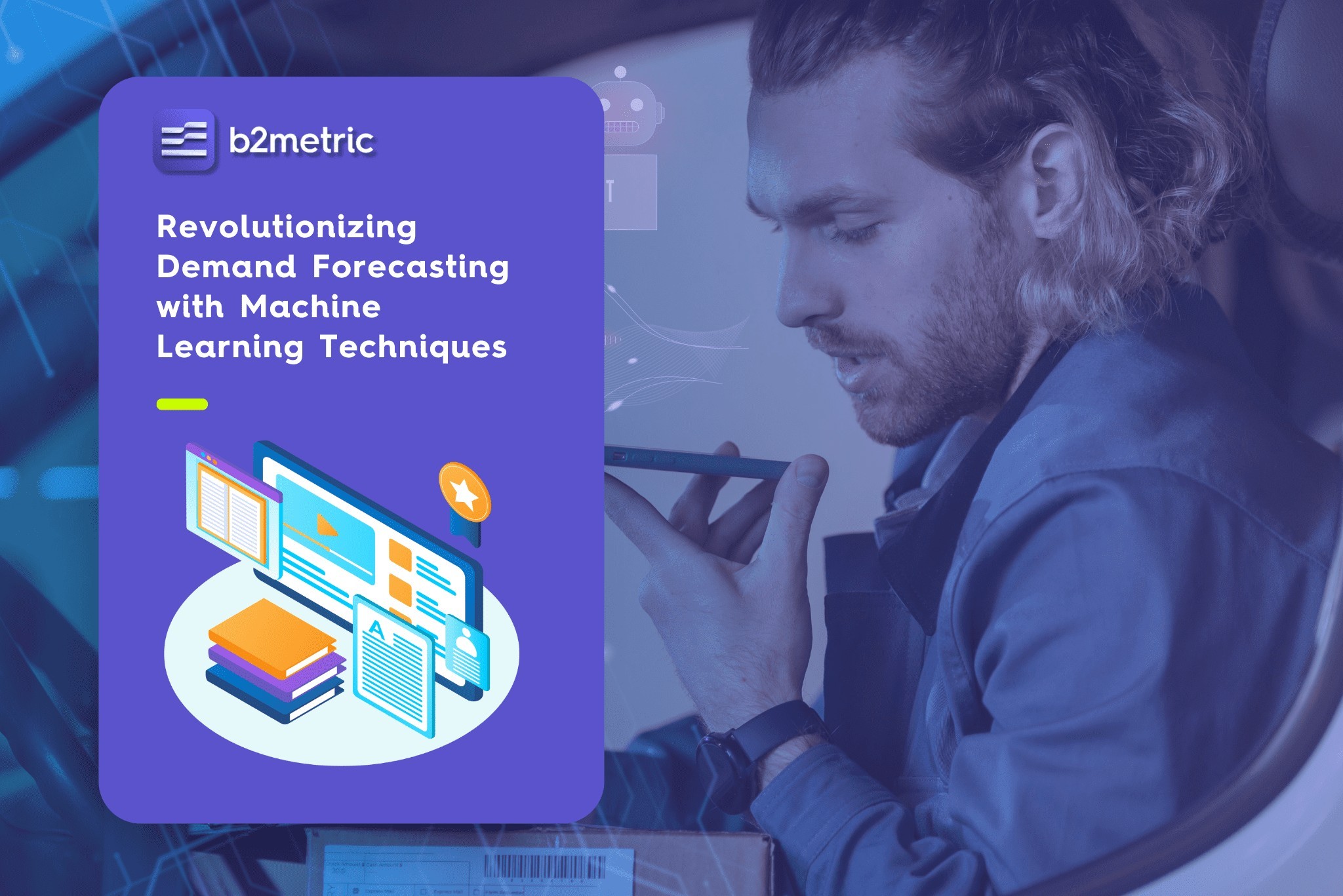
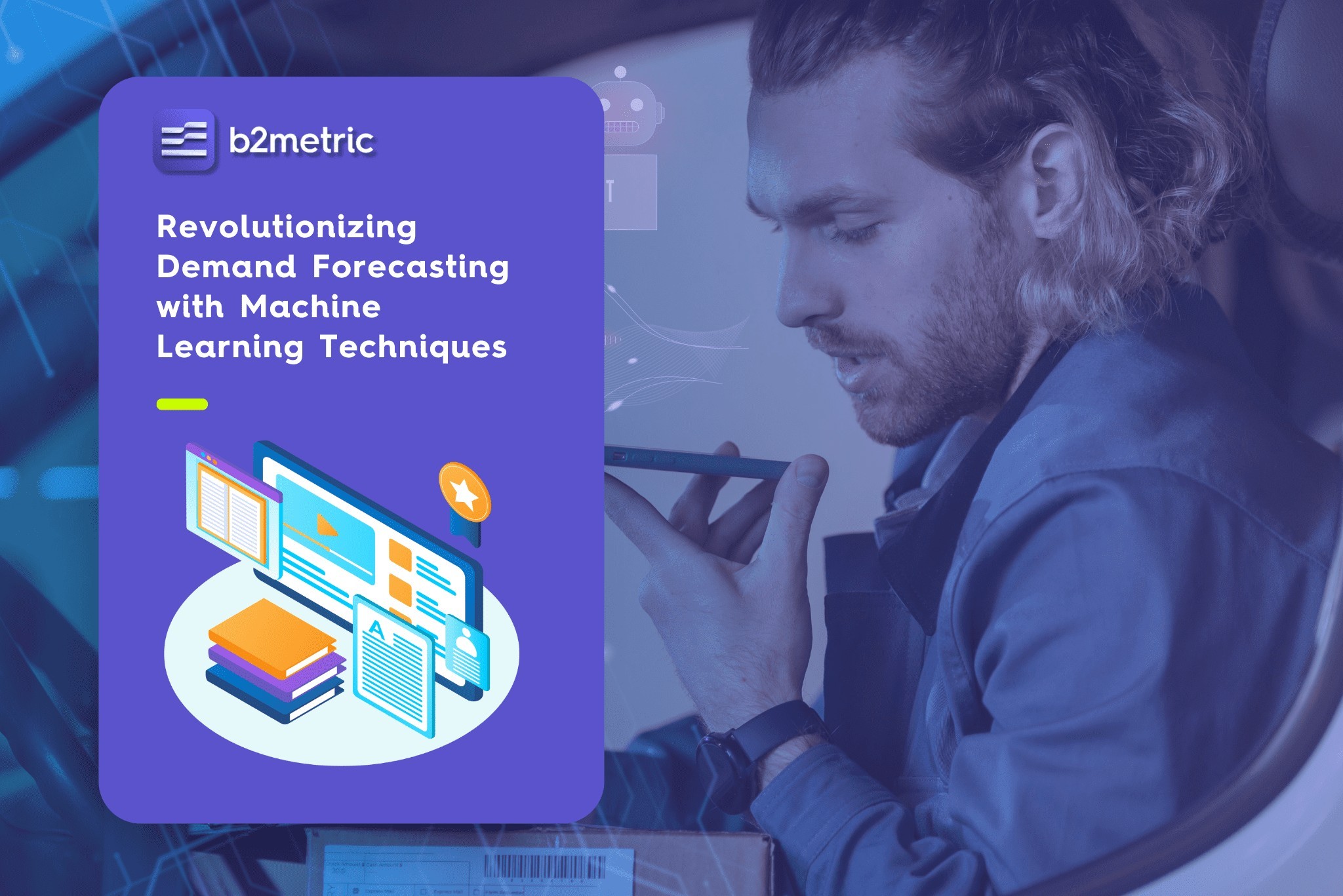
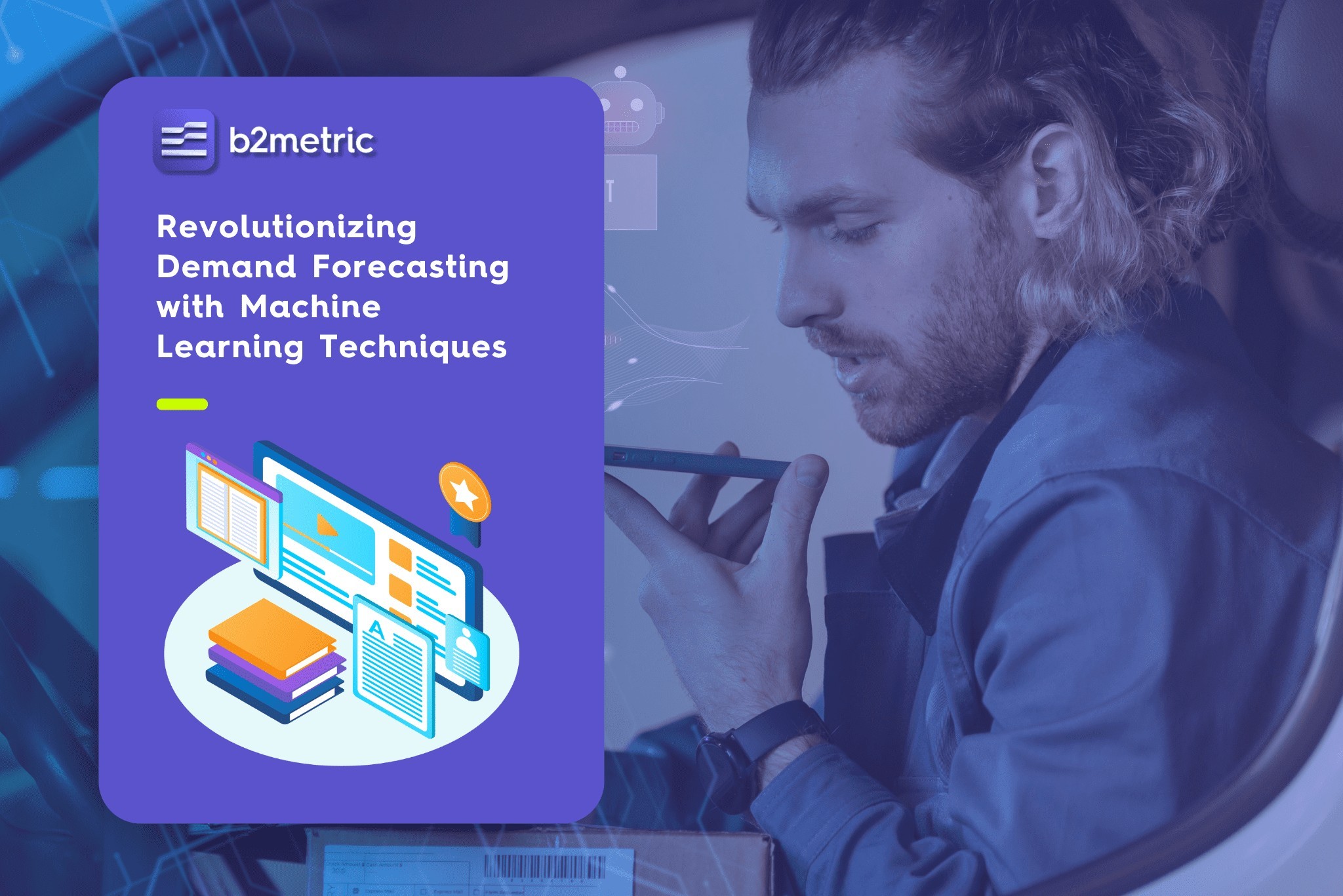
Table of contents
Table of Content
Demand Forecasting
What Are the Various Demand Forecasting Methods?
AI & ML for Retailers
Demand Forecasting for Retail
As B2Metric, we enable companies to forecast demand with higher accuracy. In addition to traditional statistical methods, we use an automated machine learning pipeline that detects the effect of external factors affecting the market through machine learning.
Demand Forecasting
Fluctuations in demands constitute one of the biggest problems of company managers. Many factors, from the weather to social media posts, cause consumers to change their buying behavior frequently. Moreover, these changes are experienced over a short period but briefly. For all these reasons, it becomes increasingly challenging to anticipate consumers' demands and follow a path accordingly in production.
With businesses in almost every sector facing increasing demand fluctuations and rapidly developing market conditions, advanced demand forecasting methods are more important than ever. With demand forecasting, companies can make better product and target market decisions. On the other hand, wrong choices can adversely affect stock holding costs, customer satisfaction, supply chain management, and profitability. In summary, you must make demand planning and sales forecasting to create an effective production process. There are different demand forecasting methods you can apply for this.

What Are the Various Demand Forecasting Methods?
Qualified Estimation Method
Qualified forecasting techniques are one of the demand forecasting methods used when there is not much data to work on, like new businesses, or when a new product is launched. In this case, various techniques such as expert opinion, market research, and comparative analysis are used to generate quantitative predictions about consumer demand. This method is often used in industries such as technology where customer demand is difficult to measure in advance.
Time Series Analysis
When historical data and trends for a product or product line are precise, businesses tend to use the time series analysis approach for demand forecasting. Time series analysis effectively determines seasonal fluctuations in demand, cyclical patterns, and critical sales trends. Time series analysis is mainly used by well-established businesses with several years of data to work best and relatively stable trend patterns.
Causal Model Method
The causal model is the most sophisticated demand forecasting tool for businesses because this method requires specific information, such as the relationships between variables that affect the demand in the market, competitors, economic forces, and other socioeconomic factors. As with time series analysis, historical data is vital in creating a causal model prediction. For example, an ice cream business can create a causal model forecast by looking at past sales data, marketing budget, promotional activities, new ice cream shops in their region, competitors' prices, weather, and public demand.
Machine Learning Assisted Forecasting
We ensure you reach the highest accuracy prediction results by choosing the best model for each component among machine learning, deep learning, and statistical methods. Different scenario types are created according to changing conditions, and the most accurate strategy is produced by analyzing their effects on the estimation results. Higher accuracy estimation results are obtained by formulating internal and external factors that may affect the demands.

AI & ML for Retailers
Suppose we look at what artificial intelligence does regarding demand forecasting, evaluations, and predictions made with artificial intelligence change how a company evaluates the data in its possession. In addition, it saves time by performing processes that take a lot of time when done manually, such as data extraction, in much shorter times.
Artificial intelligence algorithms evaluate the data obtained from customers while making sales forecasts. Assuming that sales are made over the internet, the algorithm is written, the number of page views, the number of views on the search engine (Google, Mozilla, Internet Explorer, etc.), the number of pictures of the members, sales prices, and demand changes according to price changes.

Demand Forecasting for Retail
Weekdays, seasonality, and other recurring demand patterns
With machine learning, it's possible to capture the effect when multiple factors are combined—weather and day of the week. For example, demand for barbecue products increases when sunny and warm weather coincides with a weekend.
Weather, local events, and other external factors impacting sales
External factors such as competitor price changes, local concerts, and weather can substantially affect demand but are baffling to take into account in forecasts without a system that automates a big part of the work. However, at a high level, the effect can be insightful. For example, on a warm day, you'll likely see an increase in ice cream demand, whereas the rainy season will have increased sales for umbrellas.
Unknown factors impacting demand
In brick-and-mortar retail, local occurrences—such as a direct competitor opening or closing a nearby shop—may cause a shift in demand. Sadly, data on the influence causing this shift may not be logged into any system. In addition, retailers' internal arrangements often go unrecorded, such as including a product in a particular off-shelf display area in a store. Favorably, machine learning can help in these cases. Machine learning algorithms can conditionally place a "changepoint" in the forecasting model, and then track the following data to disprove or validate the hypothesis. This allows forecasts to adapt rapidly and automatically to new demand levels.
Some examples of the unknown factors impacting demand:
-Lost Deals Correction: Oversees and reports missed deals and stock-outs; merges this information into demand forecasting for more accurate evaluations of future demand.
-Product Segmentation: Segment your products by volume, sale, and lifecycle standing and establish forecasting strategies adapted for each segment.
-Store Clusters: Classify stores that are similar to each other depending on well-known store components and observed demand review.

As B2Metric, we enable companies to forecast demand with higher accuracy. For this, in addition to traditional statistical methods, we use artificial intelligence algorithms that detect the effect of external factors affecting demand through machine learning. To learn more about these solutions, sign up.
Table of Content
Demand Forecasting
What Are the Various Demand Forecasting Methods?
AI & ML for Retailers
Demand Forecasting for Retail
As B2Metric, we enable companies to forecast demand with higher accuracy. In addition to traditional statistical methods, we use an automated machine learning pipeline that detects the effect of external factors affecting the market through machine learning.
Demand Forecasting
Fluctuations in demands constitute one of the biggest problems of company managers. Many factors, from the weather to social media posts, cause consumers to change their buying behavior frequently. Moreover, these changes are experienced over a short period but briefly. For all these reasons, it becomes increasingly challenging to anticipate consumers' demands and follow a path accordingly in production.
With businesses in almost every sector facing increasing demand fluctuations and rapidly developing market conditions, advanced demand forecasting methods are more important than ever. With demand forecasting, companies can make better product and target market decisions. On the other hand, wrong choices can adversely affect stock holding costs, customer satisfaction, supply chain management, and profitability. In summary, you must make demand planning and sales forecasting to create an effective production process. There are different demand forecasting methods you can apply for this.

What Are the Various Demand Forecasting Methods?
Qualified Estimation Method
Qualified forecasting techniques are one of the demand forecasting methods used when there is not much data to work on, like new businesses, or when a new product is launched. In this case, various techniques such as expert opinion, market research, and comparative analysis are used to generate quantitative predictions about consumer demand. This method is often used in industries such as technology where customer demand is difficult to measure in advance.
Time Series Analysis
When historical data and trends for a product or product line are precise, businesses tend to use the time series analysis approach for demand forecasting. Time series analysis effectively determines seasonal fluctuations in demand, cyclical patterns, and critical sales trends. Time series analysis is mainly used by well-established businesses with several years of data to work best and relatively stable trend patterns.
Causal Model Method
The causal model is the most sophisticated demand forecasting tool for businesses because this method requires specific information, such as the relationships between variables that affect the demand in the market, competitors, economic forces, and other socioeconomic factors. As with time series analysis, historical data is vital in creating a causal model prediction. For example, an ice cream business can create a causal model forecast by looking at past sales data, marketing budget, promotional activities, new ice cream shops in their region, competitors' prices, weather, and public demand.
Machine Learning Assisted Forecasting
We ensure you reach the highest accuracy prediction results by choosing the best model for each component among machine learning, deep learning, and statistical methods. Different scenario types are created according to changing conditions, and the most accurate strategy is produced by analyzing their effects on the estimation results. Higher accuracy estimation results are obtained by formulating internal and external factors that may affect the demands.

AI & ML for Retailers
Suppose we look at what artificial intelligence does regarding demand forecasting, evaluations, and predictions made with artificial intelligence change how a company evaluates the data in its possession. In addition, it saves time by performing processes that take a lot of time when done manually, such as data extraction, in much shorter times.
Artificial intelligence algorithms evaluate the data obtained from customers while making sales forecasts. Assuming that sales are made over the internet, the algorithm is written, the number of page views, the number of views on the search engine (Google, Mozilla, Internet Explorer, etc.), the number of pictures of the members, sales prices, and demand changes according to price changes.

Demand Forecasting for Retail
Weekdays, seasonality, and other recurring demand patterns
With machine learning, it's possible to capture the effect when multiple factors are combined—weather and day of the week. For example, demand for barbecue products increases when sunny and warm weather coincides with a weekend.
Weather, local events, and other external factors impacting sales
External factors such as competitor price changes, local concerts, and weather can substantially affect demand but are baffling to take into account in forecasts without a system that automates a big part of the work. However, at a high level, the effect can be insightful. For example, on a warm day, you'll likely see an increase in ice cream demand, whereas the rainy season will have increased sales for umbrellas.
Unknown factors impacting demand
In brick-and-mortar retail, local occurrences—such as a direct competitor opening or closing a nearby shop—may cause a shift in demand. Sadly, data on the influence causing this shift may not be logged into any system. In addition, retailers' internal arrangements often go unrecorded, such as including a product in a particular off-shelf display area in a store. Favorably, machine learning can help in these cases. Machine learning algorithms can conditionally place a "changepoint" in the forecasting model, and then track the following data to disprove or validate the hypothesis. This allows forecasts to adapt rapidly and automatically to new demand levels.
Some examples of the unknown factors impacting demand:
-Lost Deals Correction: Oversees and reports missed deals and stock-outs; merges this information into demand forecasting for more accurate evaluations of future demand.
-Product Segmentation: Segment your products by volume, sale, and lifecycle standing and establish forecasting strategies adapted for each segment.
-Store Clusters: Classify stores that are similar to each other depending on well-known store components and observed demand review.

As B2Metric, we enable companies to forecast demand with higher accuracy. For this, in addition to traditional statistical methods, we use artificial intelligence algorithms that detect the effect of external factors affecting demand through machine learning. To learn more about these solutions, sign up.
Table of Content
Demand Forecasting
What Are the Various Demand Forecasting Methods?
AI & ML for Retailers
Demand Forecasting for Retail
As B2Metric, we enable companies to forecast demand with higher accuracy. In addition to traditional statistical methods, we use an automated machine learning pipeline that detects the effect of external factors affecting the market through machine learning.
Demand Forecasting
Fluctuations in demands constitute one of the biggest problems of company managers. Many factors, from the weather to social media posts, cause consumers to change their buying behavior frequently. Moreover, these changes are experienced over a short period but briefly. For all these reasons, it becomes increasingly challenging to anticipate consumers' demands and follow a path accordingly in production.
With businesses in almost every sector facing increasing demand fluctuations and rapidly developing market conditions, advanced demand forecasting methods are more important than ever. With demand forecasting, companies can make better product and target market decisions. On the other hand, wrong choices can adversely affect stock holding costs, customer satisfaction, supply chain management, and profitability. In summary, you must make demand planning and sales forecasting to create an effective production process. There are different demand forecasting methods you can apply for this.

What Are the Various Demand Forecasting Methods?
Qualified Estimation Method
Qualified forecasting techniques are one of the demand forecasting methods used when there is not much data to work on, like new businesses, or when a new product is launched. In this case, various techniques such as expert opinion, market research, and comparative analysis are used to generate quantitative predictions about consumer demand. This method is often used in industries such as technology where customer demand is difficult to measure in advance.
Time Series Analysis
When historical data and trends for a product or product line are precise, businesses tend to use the time series analysis approach for demand forecasting. Time series analysis effectively determines seasonal fluctuations in demand, cyclical patterns, and critical sales trends. Time series analysis is mainly used by well-established businesses with several years of data to work best and relatively stable trend patterns.
Causal Model Method
The causal model is the most sophisticated demand forecasting tool for businesses because this method requires specific information, such as the relationships between variables that affect the demand in the market, competitors, economic forces, and other socioeconomic factors. As with time series analysis, historical data is vital in creating a causal model prediction. For example, an ice cream business can create a causal model forecast by looking at past sales data, marketing budget, promotional activities, new ice cream shops in their region, competitors' prices, weather, and public demand.
Machine Learning Assisted Forecasting
We ensure you reach the highest accuracy prediction results by choosing the best model for each component among machine learning, deep learning, and statistical methods. Different scenario types are created according to changing conditions, and the most accurate strategy is produced by analyzing their effects on the estimation results. Higher accuracy estimation results are obtained by formulating internal and external factors that may affect the demands.

AI & ML for Retailers
Suppose we look at what artificial intelligence does regarding demand forecasting, evaluations, and predictions made with artificial intelligence change how a company evaluates the data in its possession. In addition, it saves time by performing processes that take a lot of time when done manually, such as data extraction, in much shorter times.
Artificial intelligence algorithms evaluate the data obtained from customers while making sales forecasts. Assuming that sales are made over the internet, the algorithm is written, the number of page views, the number of views on the search engine (Google, Mozilla, Internet Explorer, etc.), the number of pictures of the members, sales prices, and demand changes according to price changes.

Demand Forecasting for Retail
Weekdays, seasonality, and other recurring demand patterns
With machine learning, it's possible to capture the effect when multiple factors are combined—weather and day of the week. For example, demand for barbecue products increases when sunny and warm weather coincides with a weekend.
Weather, local events, and other external factors impacting sales
External factors such as competitor price changes, local concerts, and weather can substantially affect demand but are baffling to take into account in forecasts without a system that automates a big part of the work. However, at a high level, the effect can be insightful. For example, on a warm day, you'll likely see an increase in ice cream demand, whereas the rainy season will have increased sales for umbrellas.
Unknown factors impacting demand
In brick-and-mortar retail, local occurrences—such as a direct competitor opening or closing a nearby shop—may cause a shift in demand. Sadly, data on the influence causing this shift may not be logged into any system. In addition, retailers' internal arrangements often go unrecorded, such as including a product in a particular off-shelf display area in a store. Favorably, machine learning can help in these cases. Machine learning algorithms can conditionally place a "changepoint" in the forecasting model, and then track the following data to disprove or validate the hypothesis. This allows forecasts to adapt rapidly and automatically to new demand levels.
Some examples of the unknown factors impacting demand:
-Lost Deals Correction: Oversees and reports missed deals and stock-outs; merges this information into demand forecasting for more accurate evaluations of future demand.
-Product Segmentation: Segment your products by volume, sale, and lifecycle standing and establish forecasting strategies adapted for each segment.
-Store Clusters: Classify stores that are similar to each other depending on well-known store components and observed demand review.

As B2Metric, we enable companies to forecast demand with higher accuracy. For this, in addition to traditional statistical methods, we use artificial intelligence algorithms that detect the effect of external factors affecting demand through machine learning. To learn more about these solutions, sign up.
Related articles

May 15, 2024
|
Marketing
Customer Journey Analytics: Powering Digital Success
In today's ever-changing digital landscape, understanding your customers is more important than ever.

May 20, 2024
|
Data
DevOps Culture
DevOps encompasses the blend of values, methodologies and technological resources that enhance an organizations capacity to deliver applications and services swiftly.
FAQ
How Does B2Metric’s AI-Based CDP Work?
What Are the Advantages of Using an AI-Based CDP?
How Can B2Metric’s AI-Based CDP Help You Understand Customer Behavior?
How Does B2Metric’s AI-Based CDP Work?
What Are the Advantages of Using an AI-Based CDP?
How Can B2Metric’s AI-Based CDP Help You Understand Customer Behavior?
How Does B2Metric’s AI-Based CDP Work?
What Are the Advantages of Using an AI-Based CDP?
How Can B2Metric’s AI-Based CDP Help You Understand Customer Behavior?
How Does B2Metric’s AI-Based CDP Work?
What Are the Advantages of Using an AI-Based CDP?
How Can B2Metric’s AI-Based CDP Help You Understand Customer Behavior?
Related Blogs
Related Blogs
Related Blogs
Related Blogs
Dec 3, 2024
Hyper-Personalization in Banking: Meeting Customers Where They Are
Hyper-Personalization in Banking: Meeting Customers Where They Are
Nov 25, 2024
How Predictive Analytics is Revolutionizing the Insurance Industry?
How Predictive Analytics is Revolutionizing the Insurance Industry?
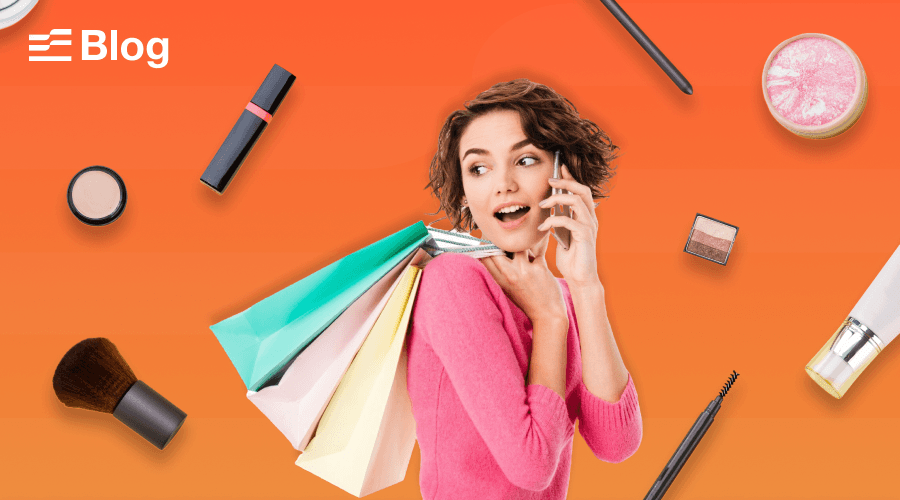
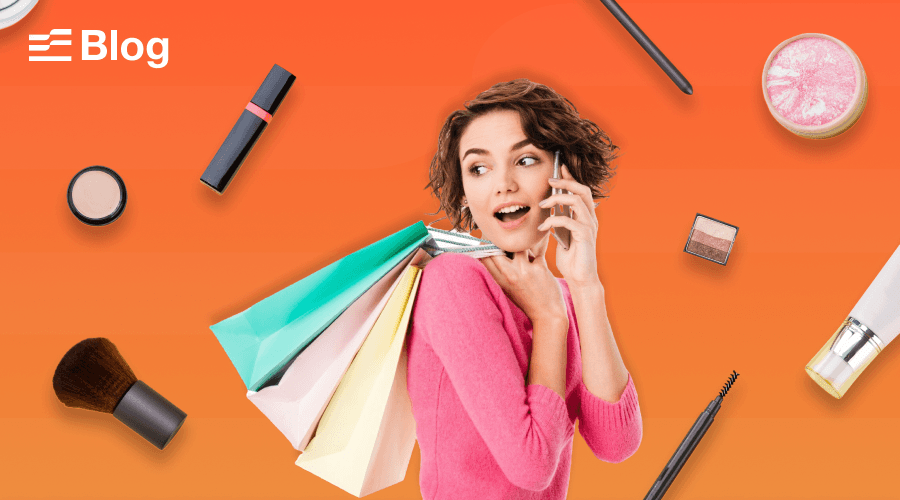
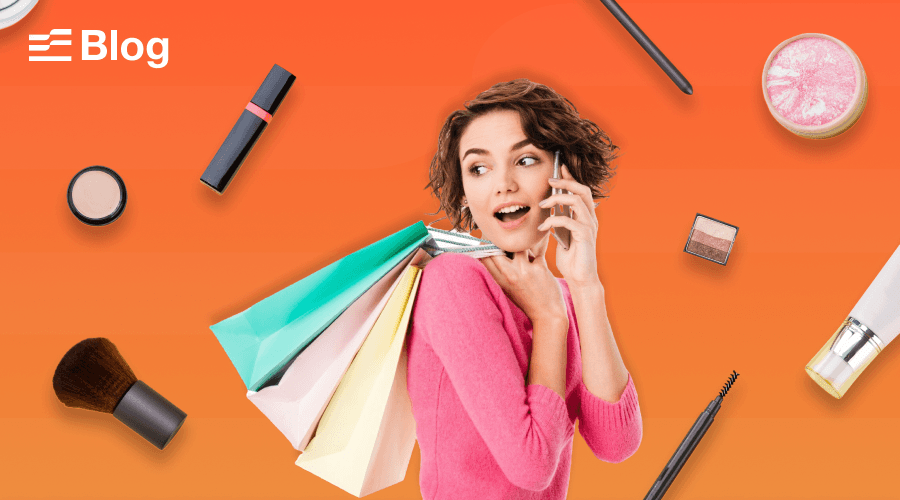
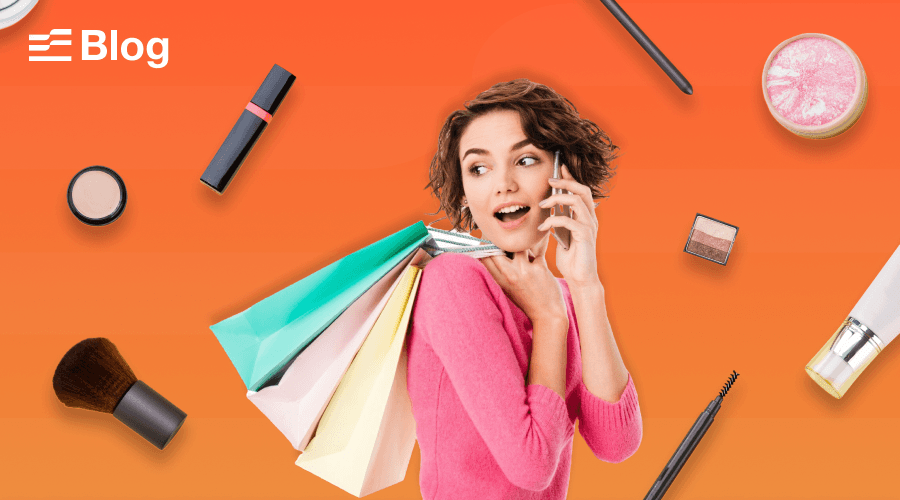
Nov 19, 2024
Maximize Customer Retention on Cyber Monday 2024: Proven Strategies
Maximize Customer Retention on Cyber Monday 2024: Proven Strategies
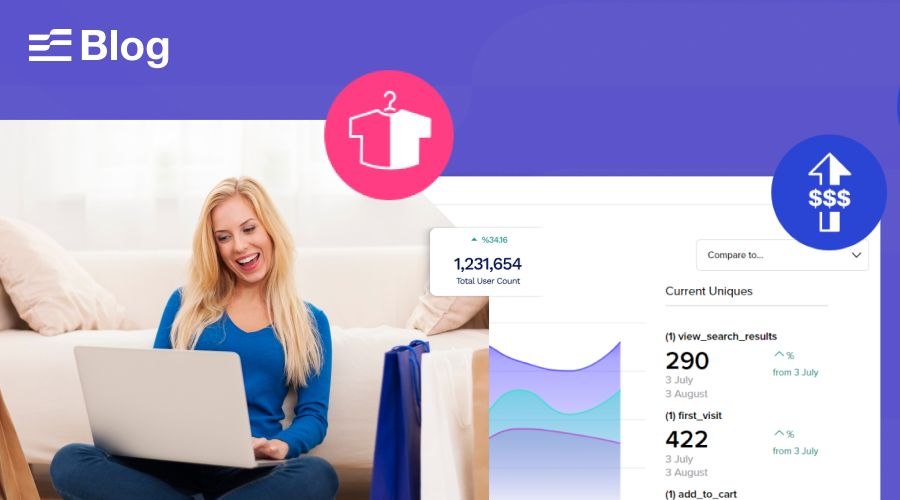
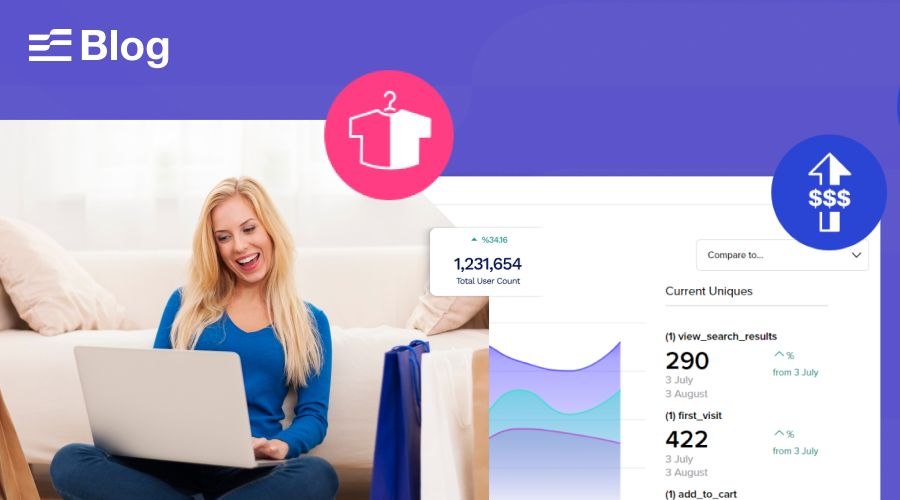
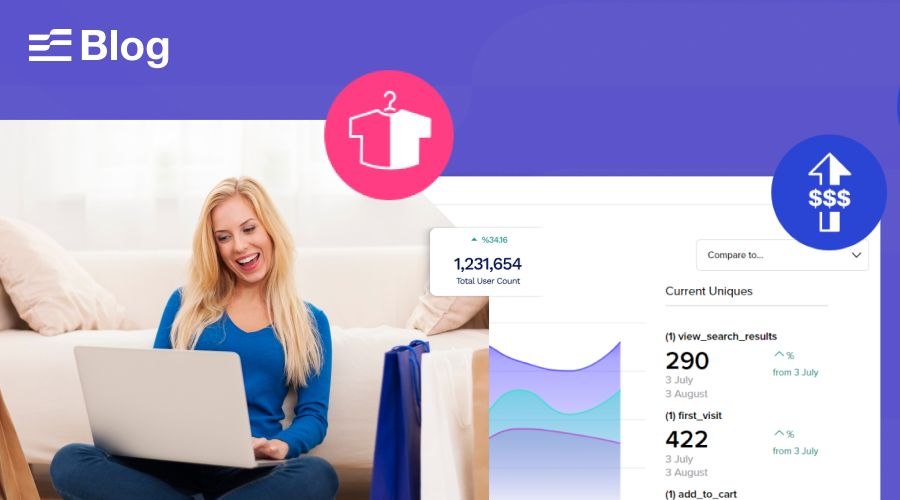
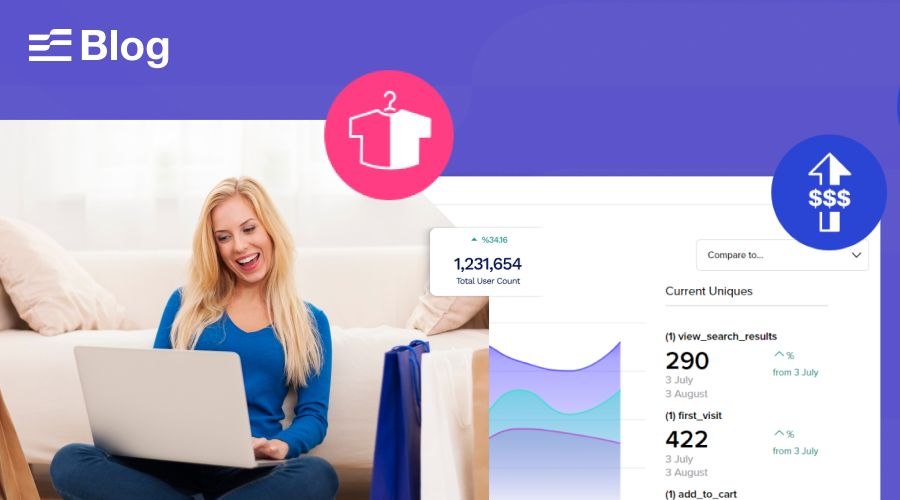
Nov 15, 2024
Predictive Analysis for Black Friday 2024: What You Need to Know
Predictive Analysis for Black Friday 2024: What You Need to Know
Product
Resources
Copyright © 2025 B2Metric | All Rights Reserved
Product
Resources
Copyright © 2025 B2Metric | All Rights Reserved
Customer intelligence data platform that helps brands analyze and predict user behavior across multi-channels.
Product
Top Blogs
Subscribe to our newsletter
Get the latest from B2Metric! 👀
Product
Subscribe to our newsletter!
Copyright © 2025 B2Metric | All Rights Reserved