B2Metric Blog
B2Metric Blog
Recent Posts
Recent Posts
Oct 18, 2024
Transform Your Marketing with B2Metric's AI-Based CDP
Transform Your Marketing with B2Metric's AI-Based CDP
Transform Your Marketing with B2Metric's AI-Based CDP
Oct 14, 2024
Boosting Customer Experience in the Finance Industry
Boosting Customer Experience in the Finance Industry
Boosting Customer Experience in the Finance Industry
Oct 7, 2024
HR Analytics Solutions: Data-Driven Strategies for Human Resources
HR Analytics Solutions: Data-Driven Strategies for Human Resources
HR Analytics Solutions: Data-Driven Strategies for Human Resources
Oct 2, 2024
E-commerce Analytics: How to Analyze Data for Your Business
E-commerce Analytics: How to Analyze Data for Your Business
E-commerce Analytics: How to Analyze Data for Your Business
Get the latest from
B2Metric! 👀
Get the latest from B2Metric! 👀
Oct 18, 2024
Transform Your Marketing with B2Metric's AI-Based CDP
Oct 14, 2024
Boosting Customer Experience in the Finance Industry
Oct 7, 2024
HR Analytics Solutions: Data-Driven Strategies for Human Resources
Oct 2, 2024
E-commerce Analytics: How to Analyze Data for Your Business

Jun 27, 2024
What is A/B Testing? A Complete Guide to Optimization with Experiments
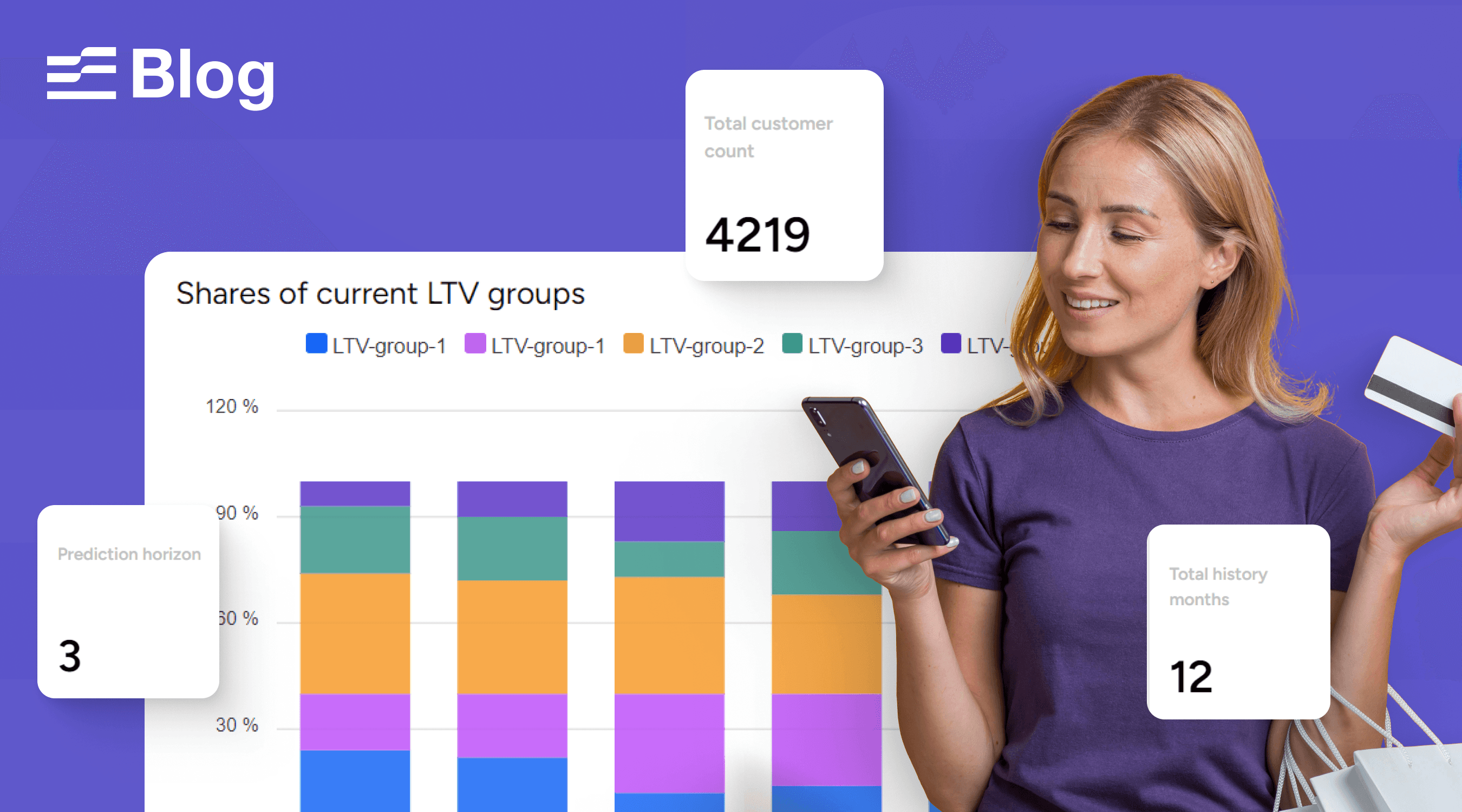
May 23, 2024
Customer Lifetime Value (LTV): How to Calculate and Maximize It
Sep 23, 2024
The Importance of Data Protection in Healthtech
Sep 18, 2024
The Importance of Funnel Analysis
Sep 16, 2024
Understanding User Behavior: Key Insights for Business Growth
Sep 11, 2024
Why Segmentation is Important in Marketing: Key Benefits and Strategies
Sep 2, 2024
Transforming Retail and E-commerce with Product Analytics: Key Trends and Strategies
Aug 27, 2024
Transforming Marketing Strategies with Data-Driven Insights: The Future of Smart Campaigns
Aug 15, 2024
What is Digital Analytics?: Key Insights & Benefits
Aug 13, 2024
What is RICE Prioritization Framework?
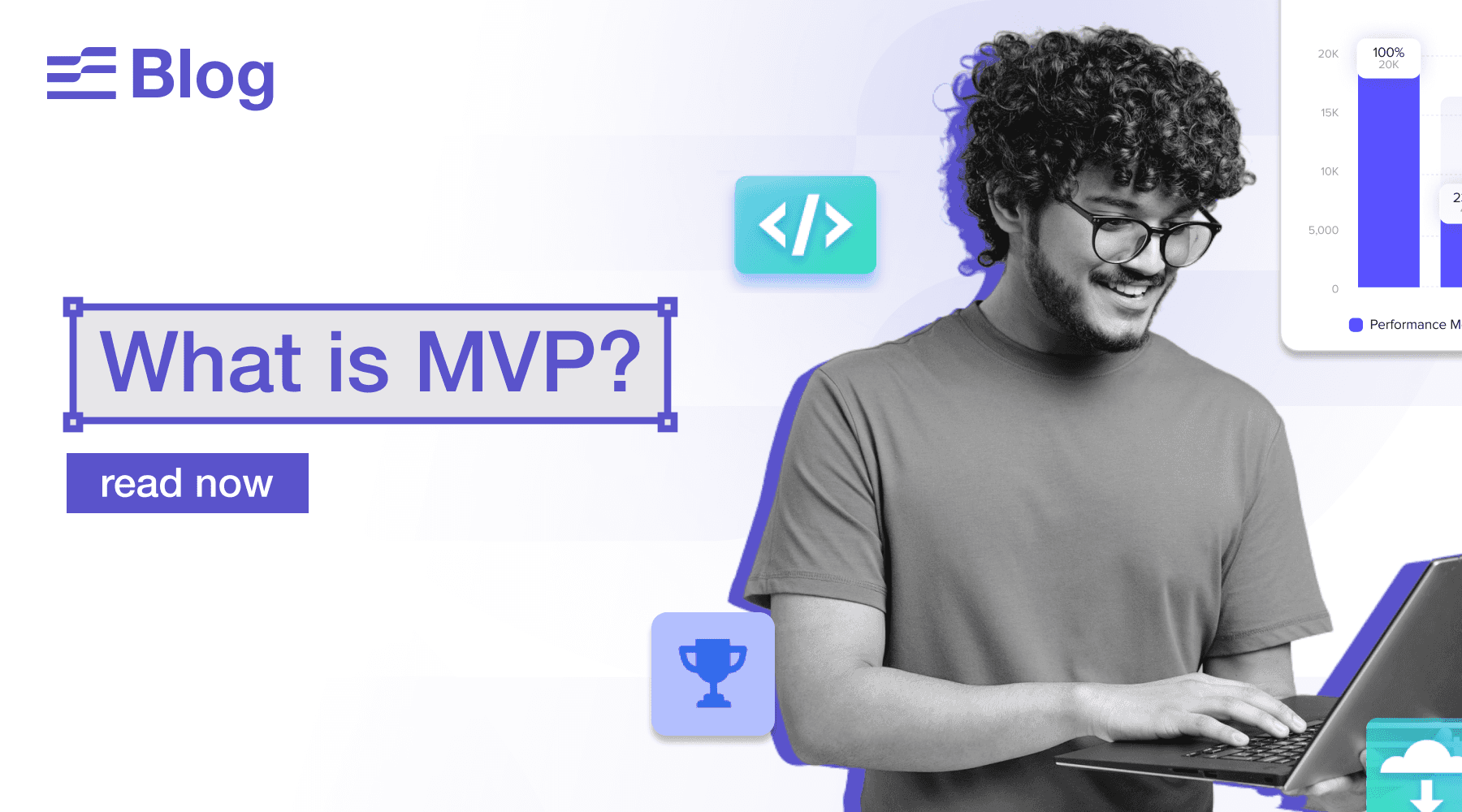
Aug 8, 2024
What is Minimum Viable Product (MVP)
Aug 6, 2024
Product Process Matrix Definition
Aug 1, 2024
What is Tech Stack? Benefits on Marketing

Jul 30, 2024
What is Lead Nurturing?
Jul 24, 2024
Sentiment Analysis: How It Works in Marketing
Jul 18, 2024
What is Marketing Attribution?

Jul 10, 2024
What is SQL? Differences Between SQL and MQL

Jul 2, 2024
What is Engagement Rate? How to Calculate Engagement Rate?
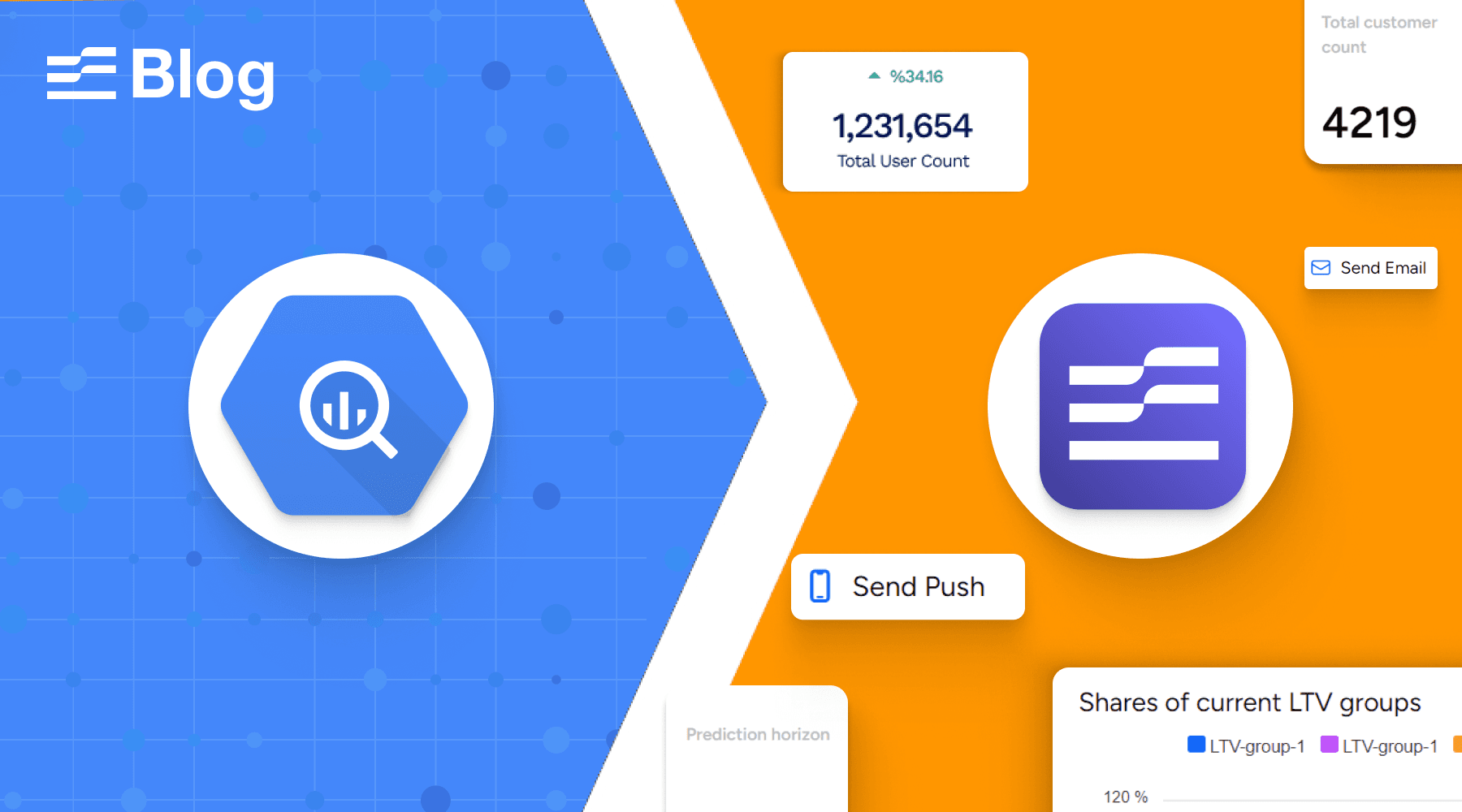
Jun 14, 2024
Why Do I Need B2metric When I Have Bigquery?
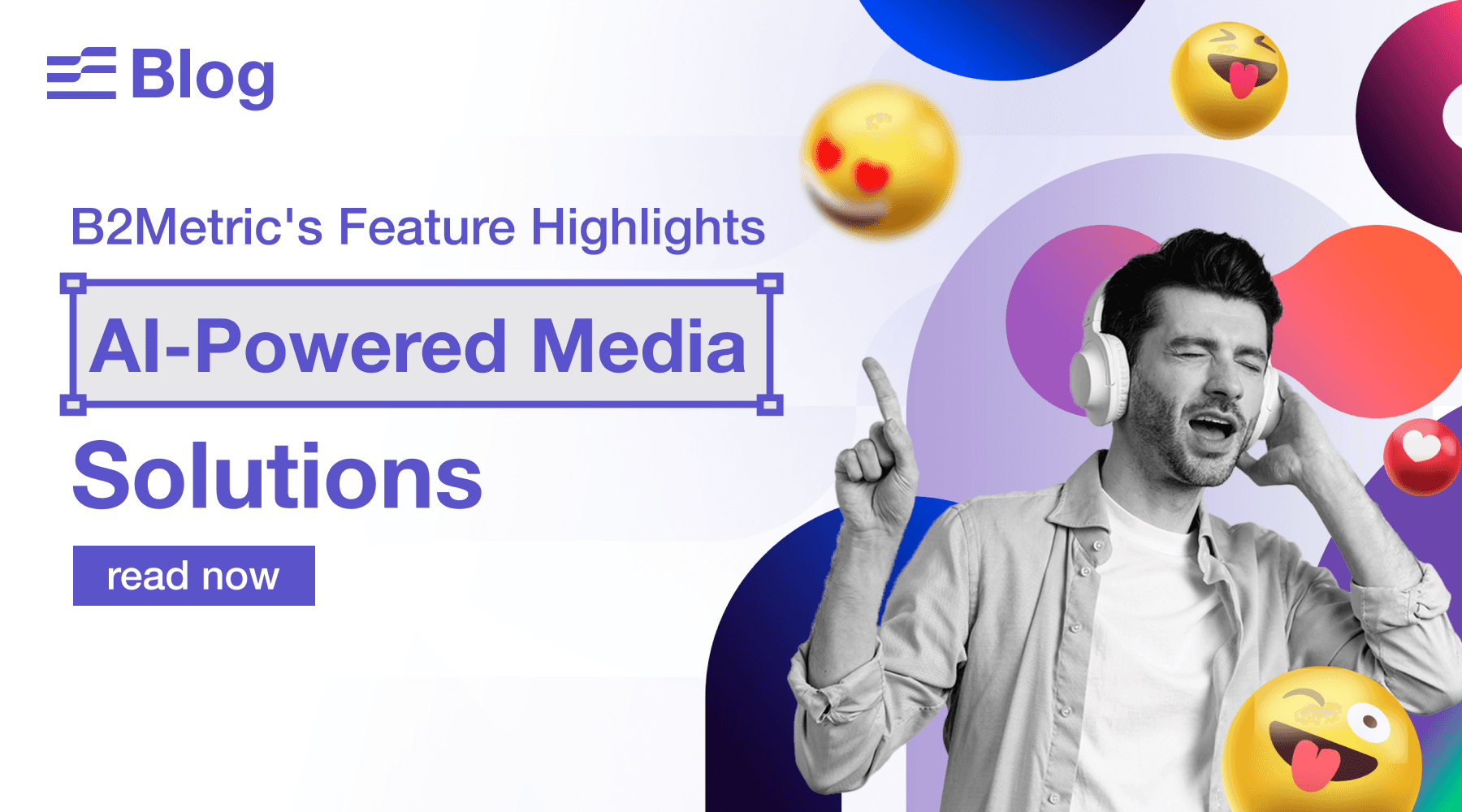
May 28, 2024
AI-Powered Media Solutions: B2Metric's Feature Highlights
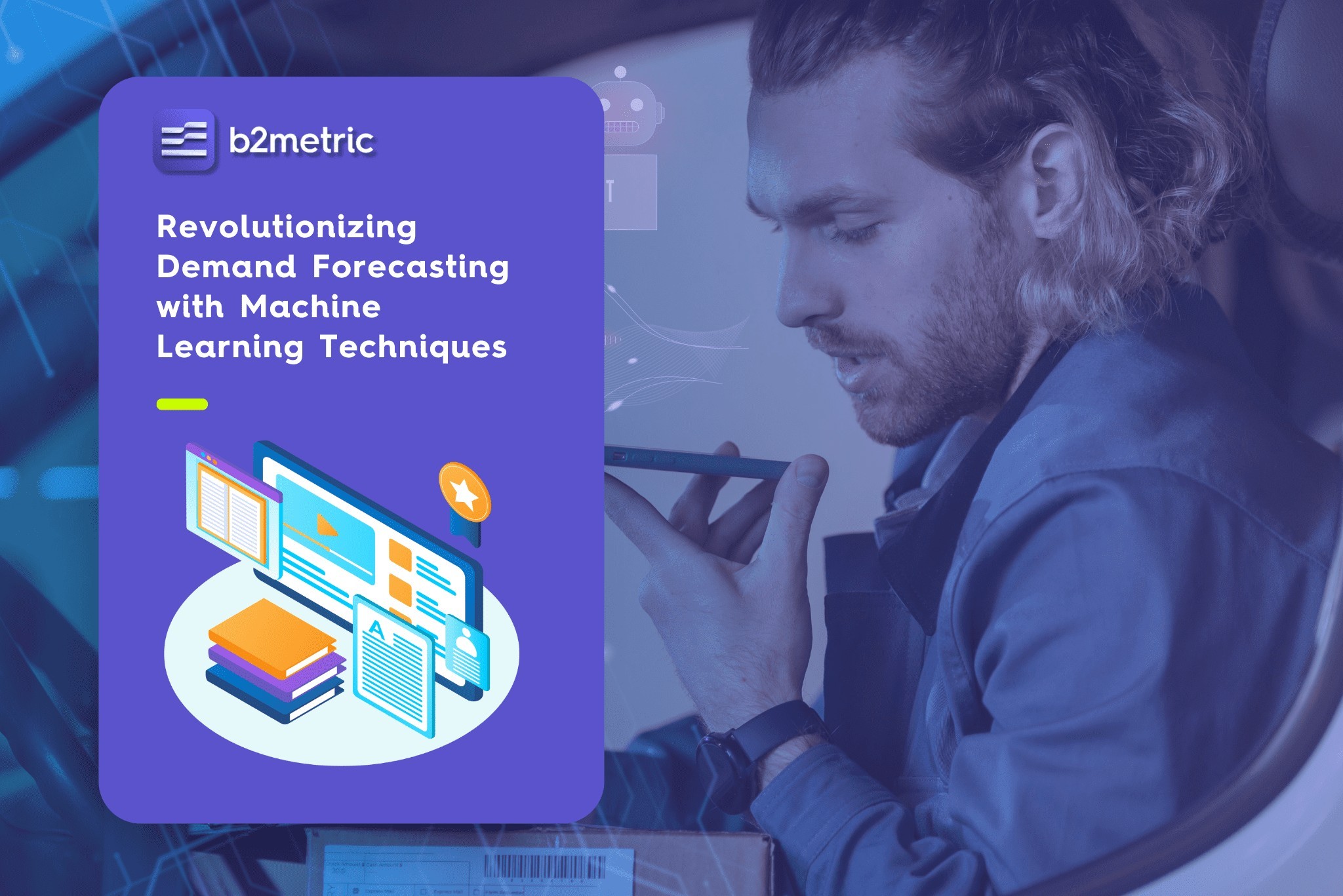
May 17, 2022
Demand Forecasting with AutoML

May 15, 2024
Customer Journey Analytics: Powering Digital Success

May 10, 2024
What is the North Star Metric?

Apr 15, 2024
What is Cross-Channel Campaign Management?

Mar 13, 2024
Insights from the Gartner Data & Analytics Summit 2024 with B2Metric

Mar 1, 2024
Database Marketing: Benefits and Strategies

Feb 9, 2024
Growth Paths in Tech: What the Heck is Product-Led Growth Marketing?

Feb 7, 2024
Measuring active and engaged users: Understanding dau/mau/wau

Feb 5, 2024
Utilizing Mobile Data Analytics for Effective Marketing Strategies

Jan 30, 2024
An Overview of Customer Journey Analytics Tools

Jan 26, 2024
Unveiling The Essence of Customer Retention and Loyalty

Jan 24, 2024
What is Customer Attrition? (Potential Causes + Strategies)

Dec 22, 2023
The Essential Guide to Customer Journey Analytics

Dec 21, 2023
Low Touch vs. High Touch Engagement Models

Oct 18, 2023
What is customer adoption and how to improve it?

Dec 13, 2023
SaaS Customer Journey Mapping

Dec 12, 2023
An Overview of Churn Management Strategies For All Businesses

Dec 7, 2023
The Benefits of Predictive Analytics in Insurance

Dec 1, 2023
How to Increase Customer Adoption?
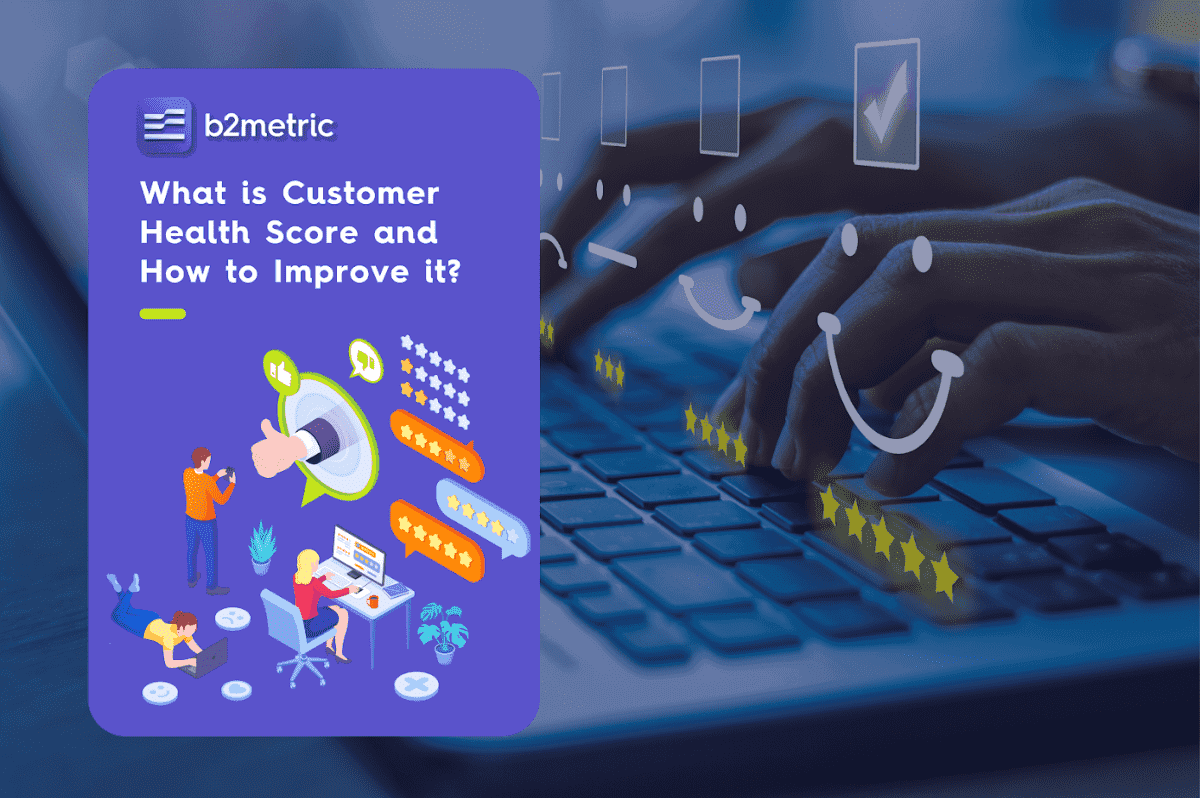
Nov 29, 2023
What is Customer Health Score and How to Imrpove it?

Nov 24, 2023
B2Metric & Turk Telekom: Transforming Campaign Optimization with Uplift Modelling

Nov 22, 2023
Machine Learning (ML) and Artificial Intelligence (AI) in Business

Nov 15, 2023
Gross Retention vs Net Retention: What is the difference?

Nov 10, 2023
11 Mobile App Analytics Metrics You Should Track for Long-term Success

Nov 7, 2023
Transforming Business Productivity with Generative AI

Nov 7, 2023
How to Avoid 3 Common Customer Data Management Mistakes in the Banking Industry?

Nov 3, 2023
Precision Targeting: The Audience Building Advantage

Oct 30, 2023
B2Metric Redefines Data Analytics: Introducing B2Metric Genii

Oct 26, 2023
The Ultimate Guide to Reduce Costs in the Aviation Industry

Sep 28, 2023
7 Strategies to Reduce Churn in the Insurance Industry

Sep 25, 2023
How to Increase Sales with Micro-Segmentation in the Pharma Industry?

May 22, 2023
A General View of Customer Loyalty and Churn Prediction

Apr 22, 2023
The Power of Predictive Analytics in Customer Behavior and Marketing Optimization
Oct 18, 2024
Transform Your Marketing with B2Metric's AI-Based CDP
Oct 14, 2024
Boosting Customer Experience in the Finance Industry
Oct 7, 2024
HR Analytics Solutions: Data-Driven Strategies for Human Resources
Oct 2, 2024
E-commerce Analytics: How to Analyze Data for Your Business

Jun 27, 2024
What is A/B Testing? A Complete Guide to Optimization with Experiments
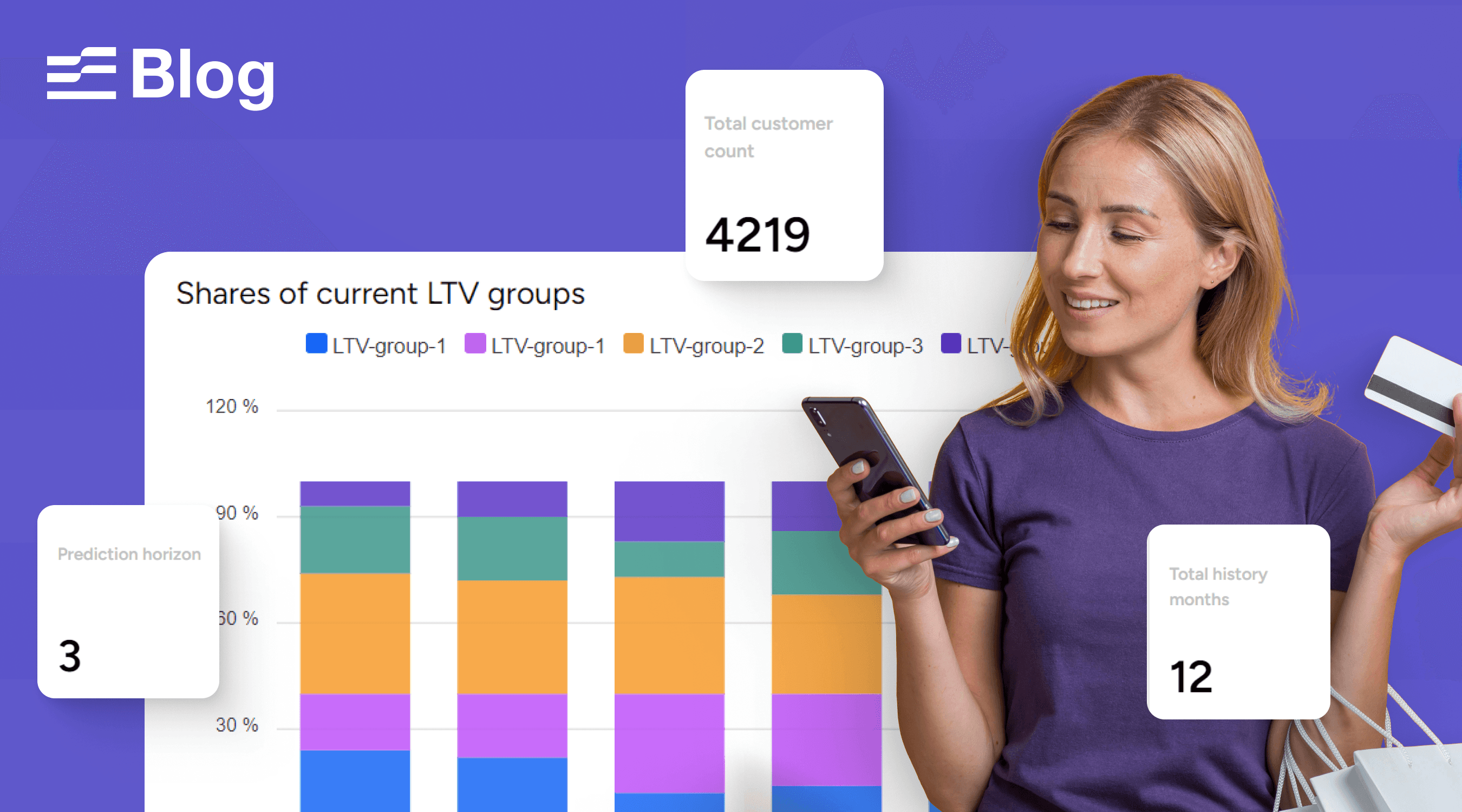
May 23, 2024
Customer Lifetime Value (LTV): How to Calculate and Maximize It
Sep 23, 2024
The Importance of Data Protection in Healthtech
Sep 18, 2024
The Importance of Funnel Analysis
Sep 16, 2024
Understanding User Behavior: Key Insights for Business Growth
Sep 11, 2024
Why Segmentation is Important in Marketing: Key Benefits and Strategies
Sep 2, 2024
Transforming Retail and E-commerce with Product Analytics: Key Trends and Strategies
Aug 27, 2024
Transforming Marketing Strategies with Data-Driven Insights: The Future of Smart Campaigns
Aug 15, 2024
What is Digital Analytics?: Key Insights & Benefits
Aug 13, 2024
What is RICE Prioritization Framework?
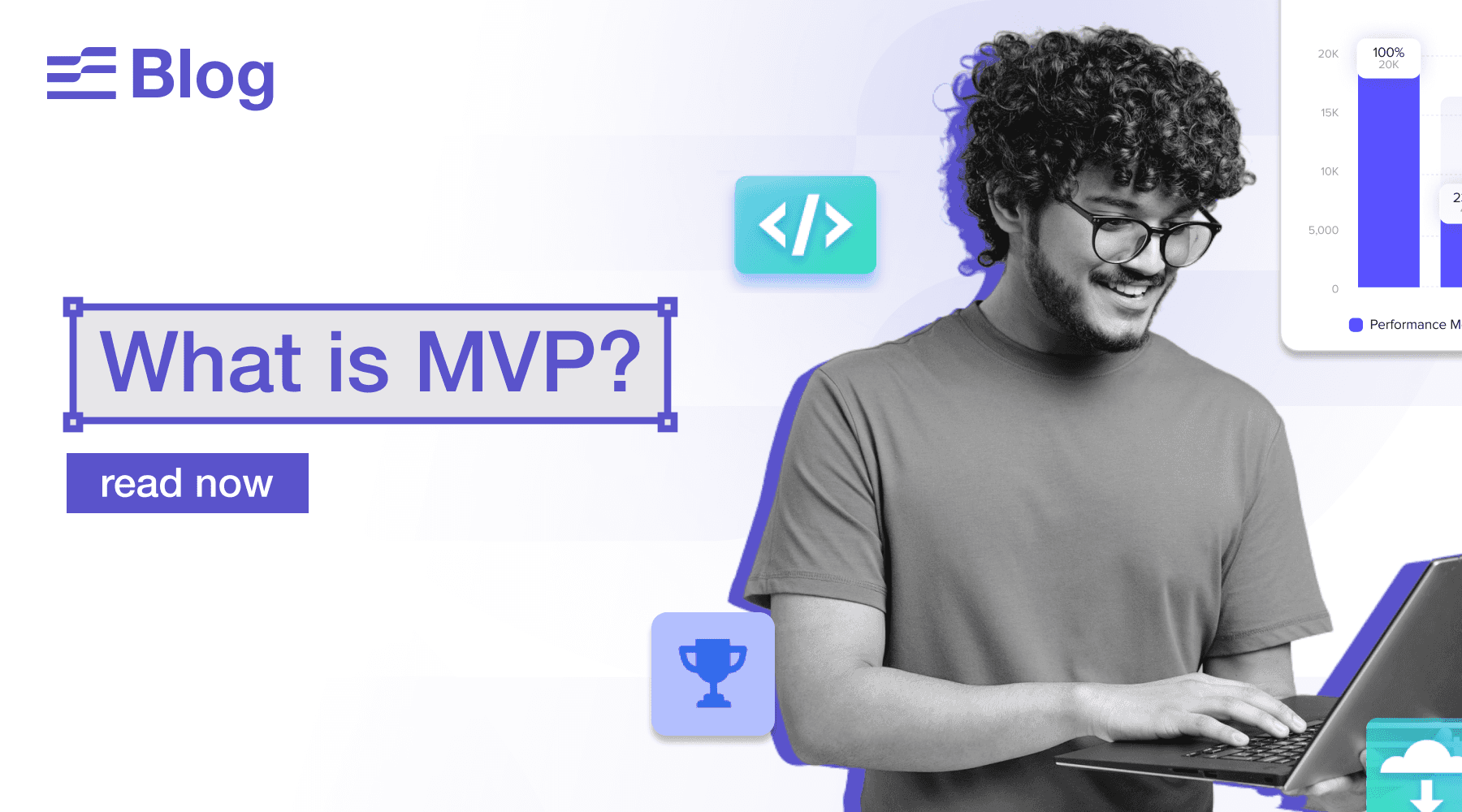
Aug 8, 2024
What is Minimum Viable Product (MVP)
Aug 6, 2024
Product Process Matrix Definition
Aug 1, 2024
What is Tech Stack? Benefits on Marketing

Jul 30, 2024
What is Lead Nurturing?
Jul 24, 2024
Sentiment Analysis: How It Works in Marketing
Jul 18, 2024
What is Marketing Attribution?

Jul 10, 2024
What is SQL? Differences Between SQL and MQL

Jul 2, 2024
What is Engagement Rate? How to Calculate Engagement Rate?
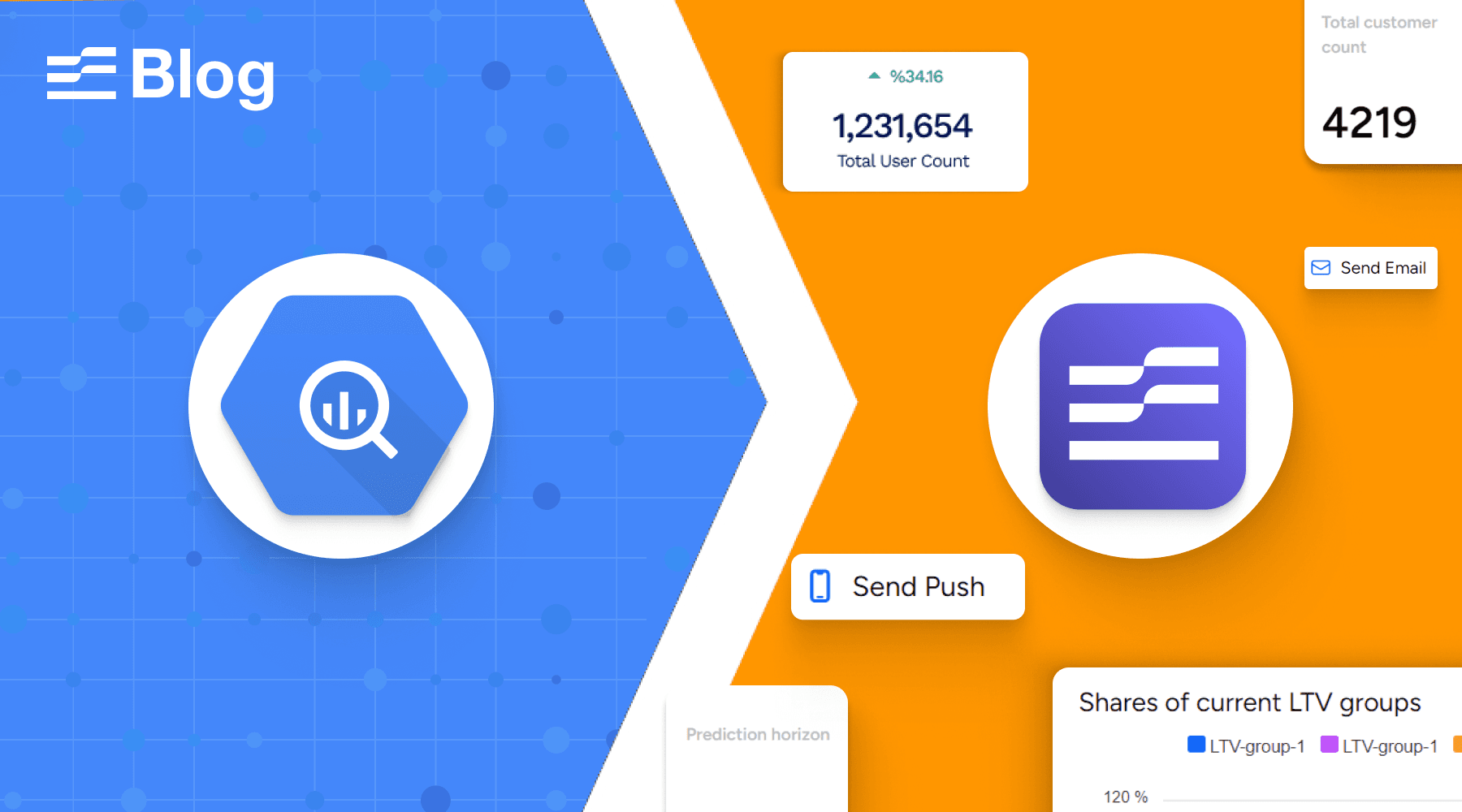
Jun 14, 2024
Why Do I Need B2metric When I Have Bigquery?
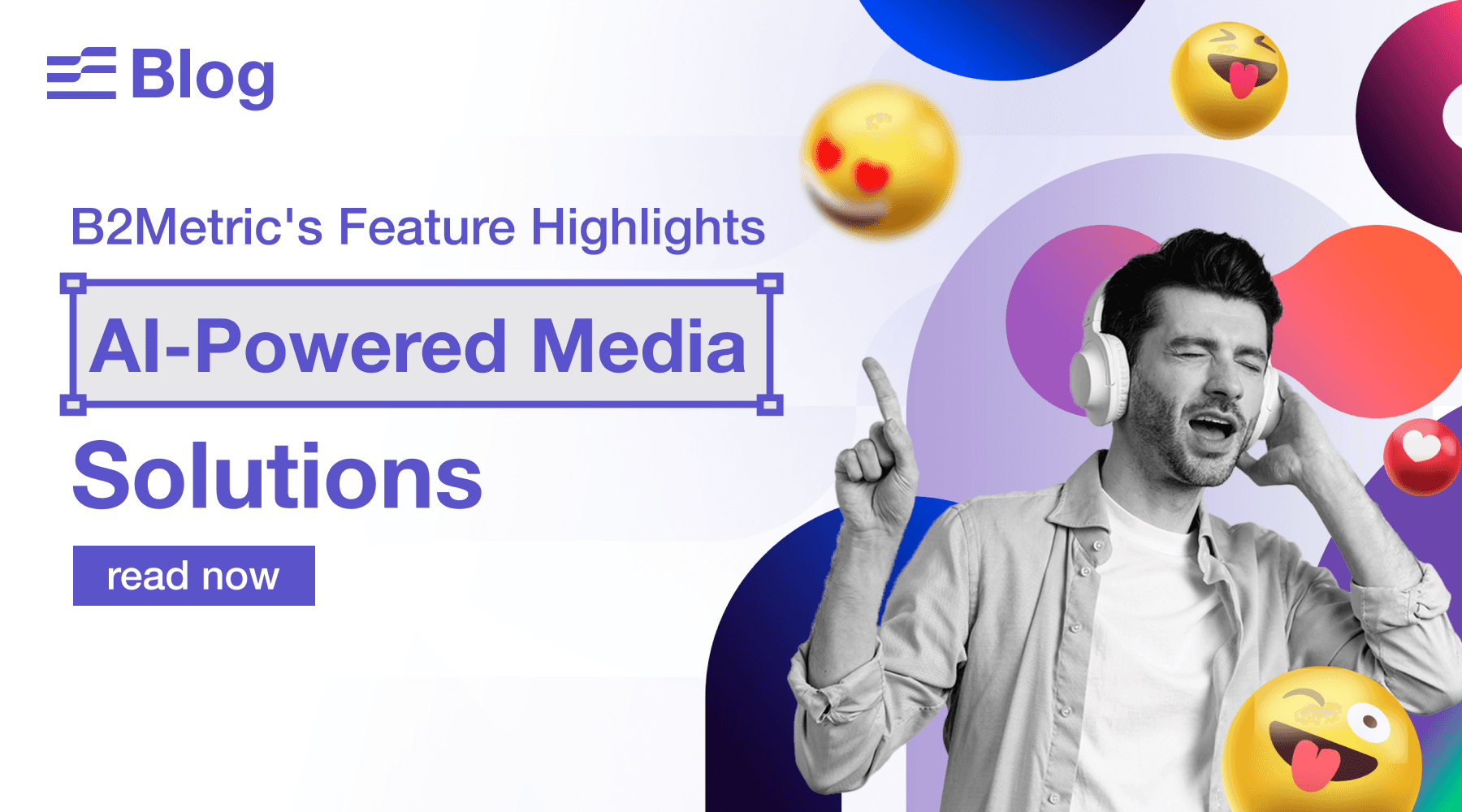
May 28, 2024
AI-Powered Media Solutions: B2Metric's Feature Highlights
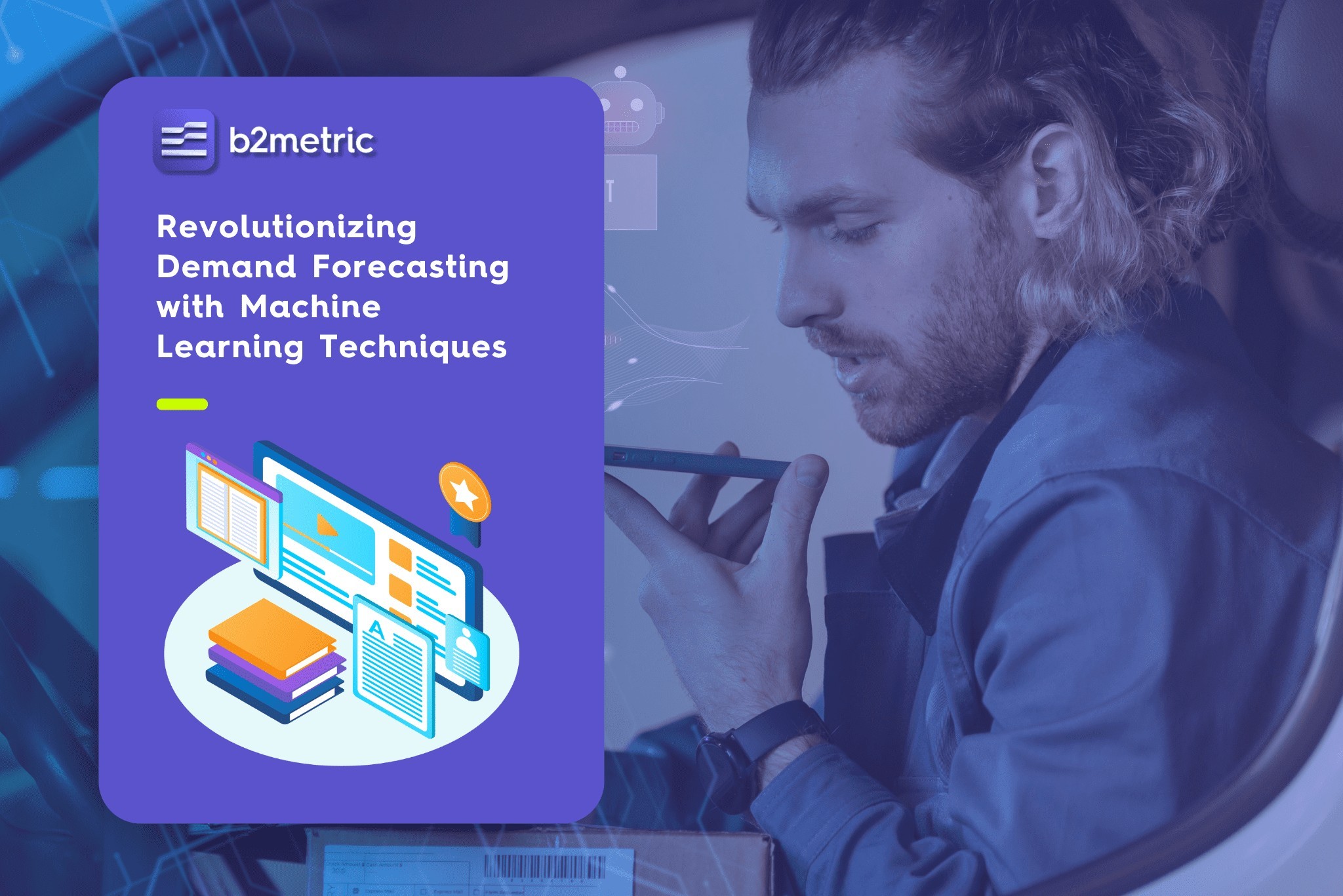
May 17, 2022
Demand Forecasting with AutoML

May 15, 2024
Customer Journey Analytics: Powering Digital Success

May 10, 2024
What is the North Star Metric?

Apr 15, 2024
What is Cross-Channel Campaign Management?

Mar 13, 2024
Insights from the Gartner Data & Analytics Summit 2024 with B2Metric

Mar 1, 2024
Database Marketing: Benefits and Strategies

Feb 9, 2024
Growth Paths in Tech: What the Heck is Product-Led Growth Marketing?

Feb 7, 2024
Measuring active and engaged users: Understanding dau/mau/wau

Feb 5, 2024
Utilizing Mobile Data Analytics for Effective Marketing Strategies

Jan 30, 2024
An Overview of Customer Journey Analytics Tools

Jan 26, 2024
Unveiling The Essence of Customer Retention and Loyalty

Jan 24, 2024
What is Customer Attrition? (Potential Causes + Strategies)

Dec 22, 2023
The Essential Guide to Customer Journey Analytics

Dec 21, 2023
Low Touch vs. High Touch Engagement Models

Oct 18, 2023
What is customer adoption and how to improve it?

Dec 13, 2023
SaaS Customer Journey Mapping

Dec 12, 2023
An Overview of Churn Management Strategies For All Businesses

Dec 7, 2023
The Benefits of Predictive Analytics in Insurance

Dec 1, 2023
How to Increase Customer Adoption?
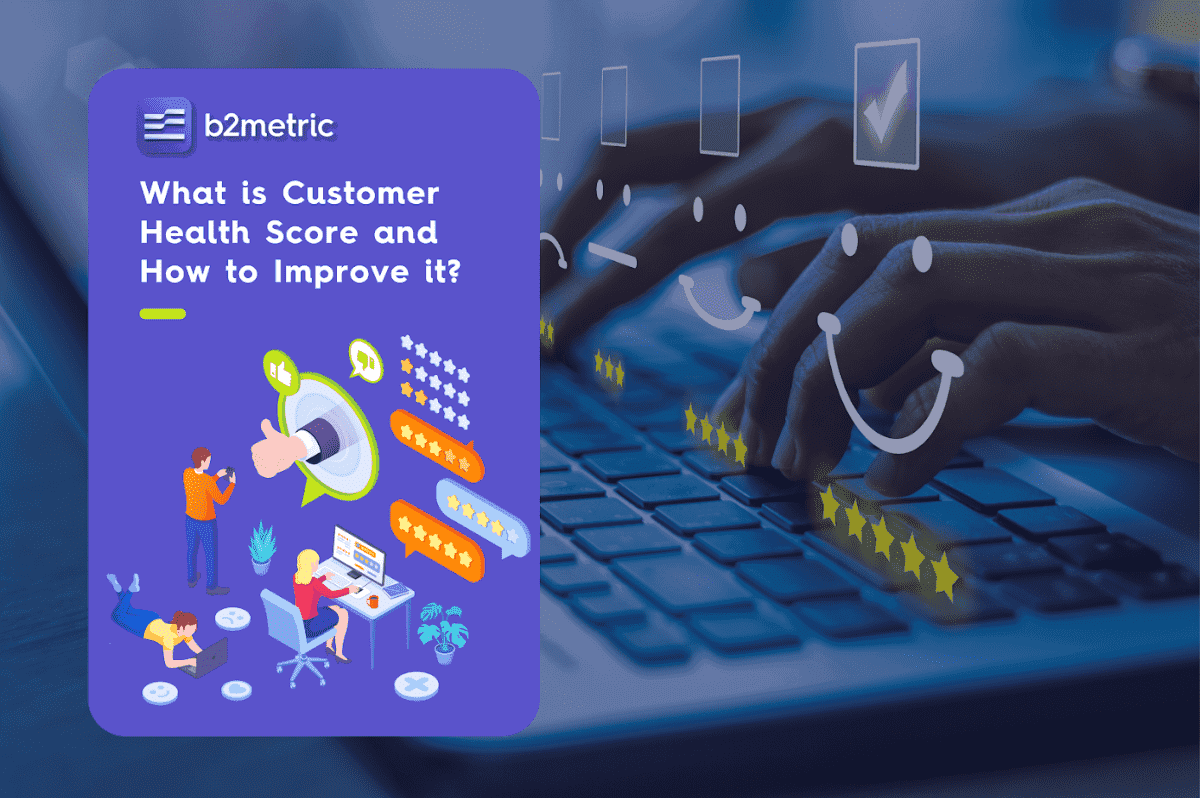
Nov 29, 2023
What is Customer Health Score and How to Imrpove it?

Nov 24, 2023
B2Metric & Turk Telekom: Transforming Campaign Optimization with Uplift Modelling

Nov 22, 2023
Machine Learning (ML) and Artificial Intelligence (AI) in Business

Nov 15, 2023
Gross Retention vs Net Retention: What is the difference?

Nov 10, 2023
11 Mobile App Analytics Metrics You Should Track for Long-term Success

Nov 7, 2023
Transforming Business Productivity with Generative AI

Nov 7, 2023
How to Avoid 3 Common Customer Data Management Mistakes in the Banking Industry?

Nov 3, 2023
Precision Targeting: The Audience Building Advantage

Oct 30, 2023
B2Metric Redefines Data Analytics: Introducing B2Metric Genii

Oct 26, 2023
The Ultimate Guide to Reduce Costs in the Aviation Industry

Sep 28, 2023
7 Strategies to Reduce Churn in the Insurance Industry

Sep 25, 2023
How to Increase Sales with Micro-Segmentation in the Pharma Industry?

May 22, 2023
A General View of Customer Loyalty and Churn Prediction

Apr 22, 2023
The Power of Predictive Analytics in Customer Behavior and Marketing Optimization
Oct 18, 2024
Transform Your Marketing with B2Metric's AI-Based CDP
Oct 14, 2024
Boosting Customer Experience in the Finance Industry
Oct 7, 2024
HR Analytics Solutions: Data-Driven Strategies for Human Resources
Oct 2, 2024
E-commerce Analytics: How to Analyze Data for Your Business

Jun 27, 2024
What is A/B Testing? A Complete Guide to Optimization with Experiments
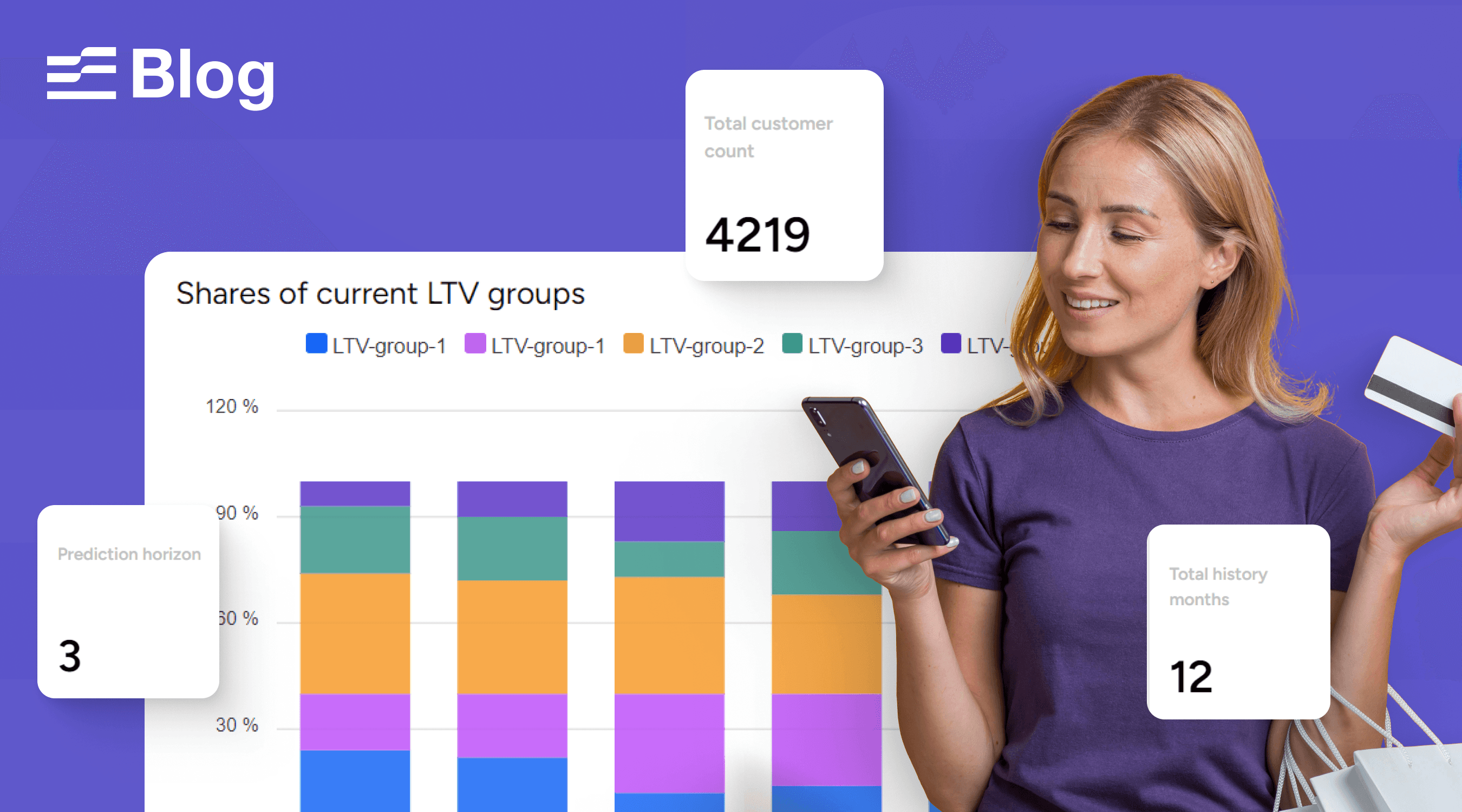
May 23, 2024
Customer Lifetime Value (LTV): How to Calculate and Maximize It
Sep 23, 2024
The Importance of Data Protection in Healthtech
Sep 18, 2024
The Importance of Funnel Analysis
Sep 16, 2024
Understanding User Behavior: Key Insights for Business Growth
Sep 11, 2024
Why Segmentation is Important in Marketing: Key Benefits and Strategies
Sep 2, 2024
Transforming Retail and E-commerce with Product Analytics: Key Trends and Strategies
Aug 27, 2024
Transforming Marketing Strategies with Data-Driven Insights: The Future of Smart Campaigns
Aug 15, 2024
What is Digital Analytics?: Key Insights & Benefits
Aug 13, 2024
What is RICE Prioritization Framework?
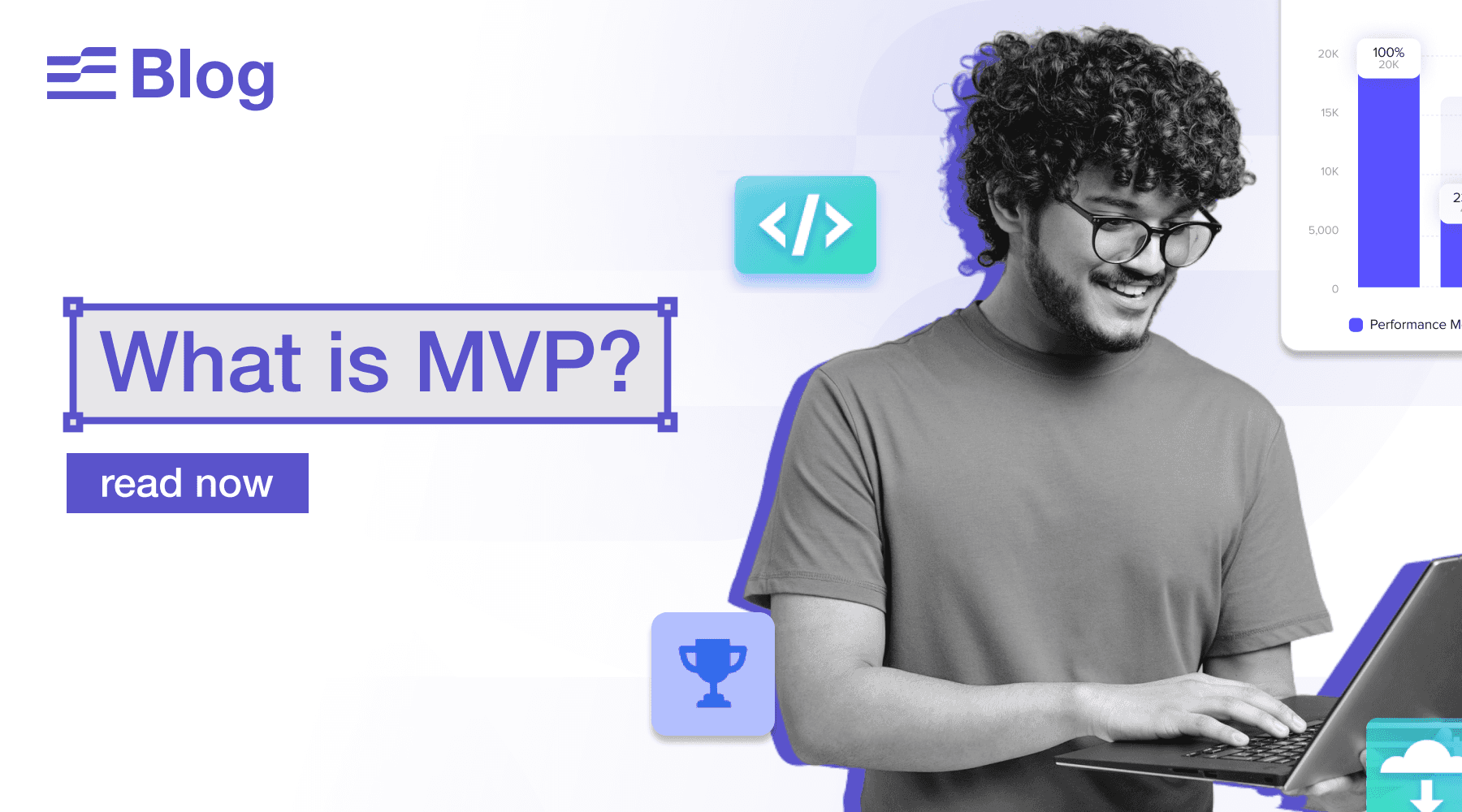
Aug 8, 2024
What is Minimum Viable Product (MVP)
Aug 6, 2024
Product Process Matrix Definition
Aug 1, 2024
What is Tech Stack? Benefits on Marketing

Jul 30, 2024
What is Lead Nurturing?
Jul 24, 2024
Sentiment Analysis: How It Works in Marketing
Jul 18, 2024
What is Marketing Attribution?

Jul 10, 2024
What is SQL? Differences Between SQL and MQL

Jul 2, 2024
What is Engagement Rate? How to Calculate Engagement Rate?
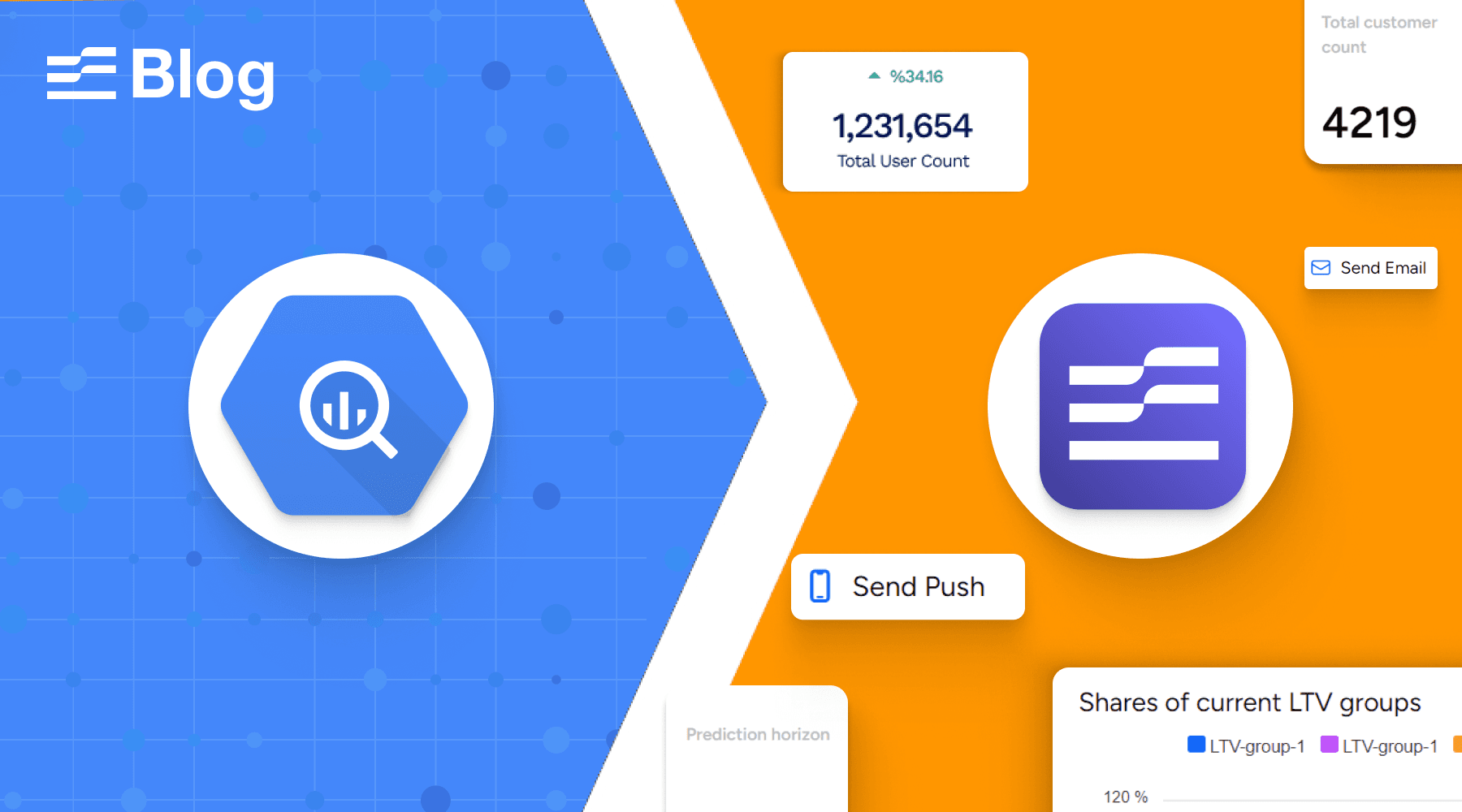
Jun 14, 2024
Why Do I Need B2metric When I Have Bigquery?
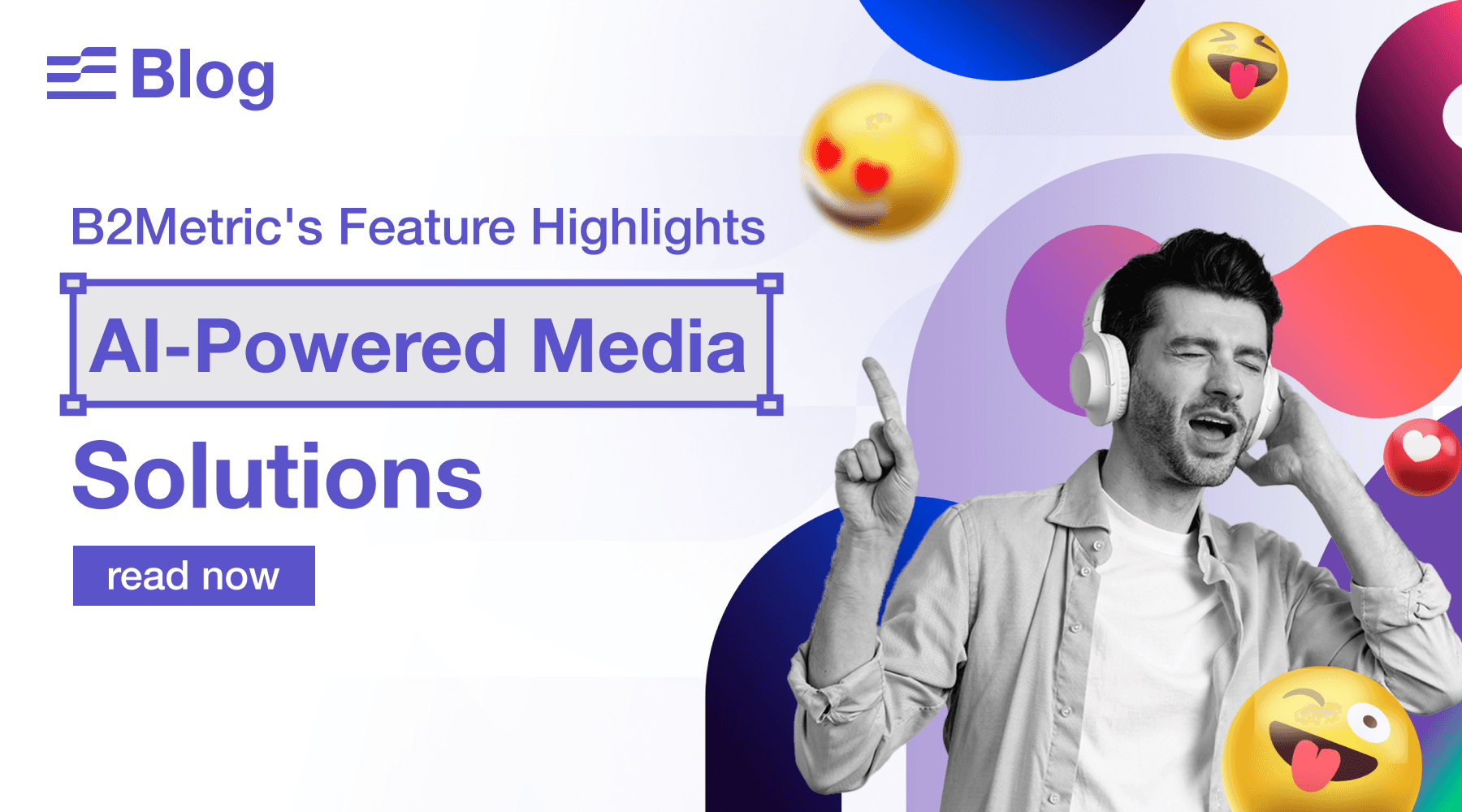
May 28, 2024
AI-Powered Media Solutions: B2Metric's Feature Highlights
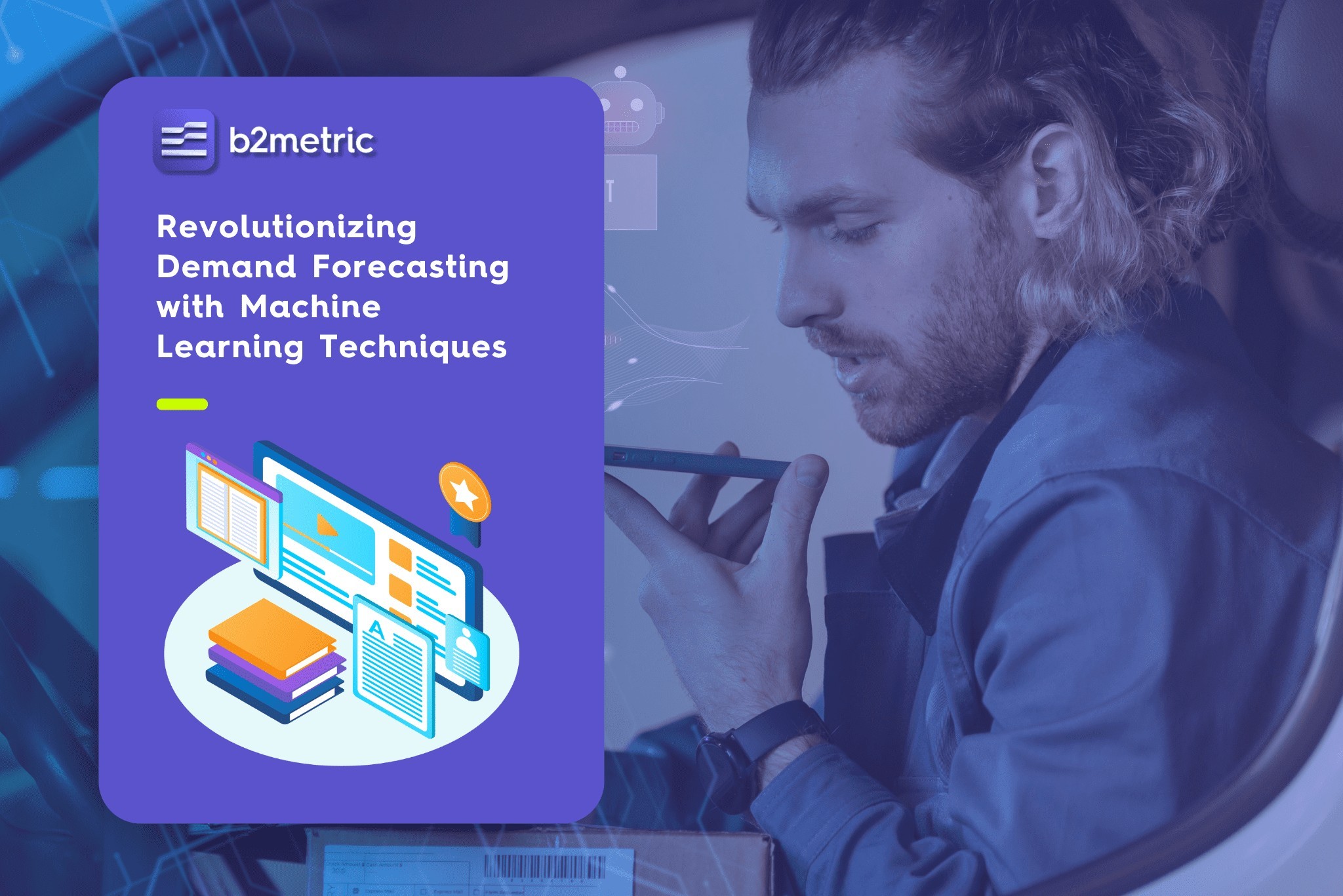
May 17, 2022
Demand Forecasting with AutoML

May 15, 2024
Customer Journey Analytics: Powering Digital Success

May 10, 2024
What is the North Star Metric?

Apr 15, 2024
What is Cross-Channel Campaign Management?

Mar 13, 2024
Insights from the Gartner Data & Analytics Summit 2024 with B2Metric

Mar 1, 2024
Database Marketing: Benefits and Strategies

Feb 9, 2024
Growth Paths in Tech: What the Heck is Product-Led Growth Marketing?

Feb 7, 2024
Measuring active and engaged users: Understanding dau/mau/wau

Feb 5, 2024
Utilizing Mobile Data Analytics for Effective Marketing Strategies

Jan 30, 2024
An Overview of Customer Journey Analytics Tools

Jan 26, 2024
Unveiling The Essence of Customer Retention and Loyalty

Jan 24, 2024
What is Customer Attrition? (Potential Causes + Strategies)

Dec 22, 2023
The Essential Guide to Customer Journey Analytics

Dec 21, 2023
Low Touch vs. High Touch Engagement Models

Oct 18, 2023
What is customer adoption and how to improve it?

Dec 13, 2023
SaaS Customer Journey Mapping

Dec 12, 2023
An Overview of Churn Management Strategies For All Businesses

Dec 7, 2023
The Benefits of Predictive Analytics in Insurance

Dec 1, 2023
How to Increase Customer Adoption?
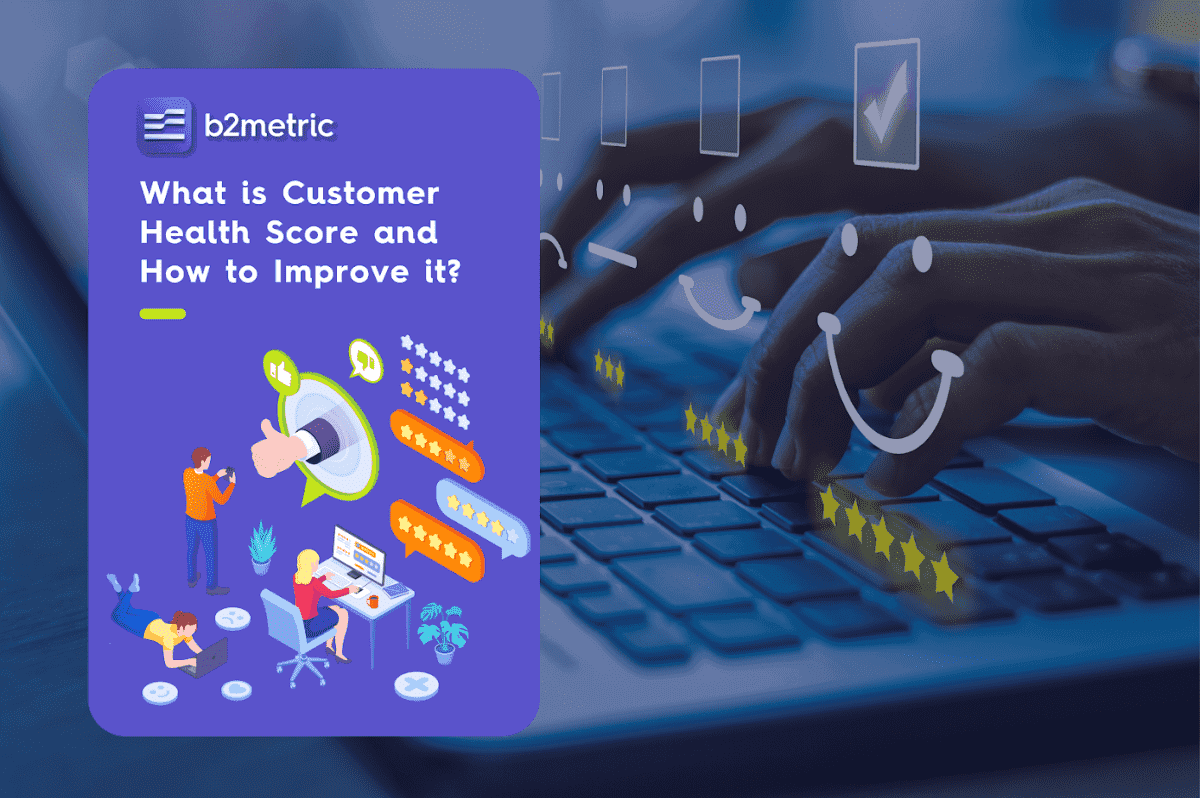
Nov 29, 2023
What is Customer Health Score and How to Imrpove it?

Nov 24, 2023
B2Metric & Turk Telekom: Transforming Campaign Optimization with Uplift Modelling

Nov 22, 2023
Machine Learning (ML) and Artificial Intelligence (AI) in Business

Nov 15, 2023
Gross Retention vs Net Retention: What is the difference?

Nov 10, 2023
11 Mobile App Analytics Metrics You Should Track for Long-term Success

Nov 7, 2023
Transforming Business Productivity with Generative AI

Nov 7, 2023
How to Avoid 3 Common Customer Data Management Mistakes in the Banking Industry?

Nov 3, 2023
Precision Targeting: The Audience Building Advantage

Oct 30, 2023
B2Metric Redefines Data Analytics: Introducing B2Metric Genii

Oct 26, 2023
The Ultimate Guide to Reduce Costs in the Aviation Industry

Sep 28, 2023
7 Strategies to Reduce Churn in the Insurance Industry

Sep 25, 2023
How to Increase Sales with Micro-Segmentation in the Pharma Industry?

May 22, 2023
A General View of Customer Loyalty and Churn Prediction

Apr 22, 2023
The Power of Predictive Analytics in Customer Behavior and Marketing Optimization
Customer intelligence data platform that helps brands analyze and predict user behavior across multi-channels.
Product
Top Blogs
Subscribe to our newsletter
Get the latest from B2Metric! 👀
Customer intelligence data platform that helps brands analyze and predict user behavior across multi-channels.
Product
Comparisons
Subscribe to our newsletter
Get the latest from B2Metric! 👀
Subscribe to our newsletter
Lorem ipsum dolor sit amet consectetur adipiscing elit aliquam mauris sed ma
Customer intelligence data platform that helps brands analyze and predict user behavior across multi-channels.
Product
Comparisons
Top Blogs
Subscribe to our newsletter
Get the latest from B2Metric! 👀