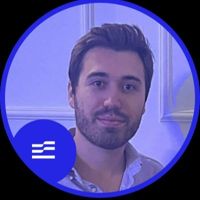
Ogulcan Ozdemir
Product Manager
|
|
|
November 25, 2024
Nov 25, 2024
Nov 25, 2024
Nov 25, 2024
Marketing and Analytics
Marketing and Analytics
Marketing and Analytics
4
4
4
4
min reading
min reading
min reading
min reading
Insurance
Insurance
Insurance
Table of contents
The Role of Predictive Analytics in Insurance
The insurance industry has always relied on data to assess risks, set premiums, and manage claims. However, with the arrival of predictive analytics, insurers can now leverage advanced technologies to make more informed decisions. By analyzing historical data and predicting future outcomes, predictive analytics is transforming how companies operate, offering accuracy and efficiency.
What is Predictive Analytics and How Does It Work?
Predictive analytics involved using statistical techniques, machine learning algorithms, and data mining to forecast future events based on historical data. In the insurance industry, it enables companies to understand patterns and assess risks.
Key Technologies Behind Predictive Analytics in Insurance
Predictive analytics relies on several technologies:
Big Data: Insurers handle massive datasets that include everything from customer demographics and driving habits to external data sources such as weather reports and economic trends. Big data technologies enable the storage, processing, and real-time analysis of these datasets, making it possible to develop more accurate and comprehensive risk assessments.
ML Models: Machine learning algorithms form the core of predictive analytics. By training on historical data, machine learning models identify patterns and relationships that would be impossible for humans to detect manually. These models continuously improve as they are exposed to more data, allowing insurers to refine their predictions, whether for assessing risk or fraudulent claims.
Telematics and IoT Devices: These technologies are impactful in auto insurance. Devices installed in vehicles and or mobile apps track driving behavior, mileage, and even accident impact data. THe real-time information feeds directly into predictive models, allowing insurers to adjust premiums based on actual behavior.
Predictive Analytics Software: Specialized software solutions integrate the above technologies into a cohesive platform. These tools provide user-friendly interfaces for insurers to analyze data, generate predictive reports, and implement strategies.
How Predictive Analytics Improve Risk Assessment
Enhancing Underwriting with Data Driven Insights
Underwriting has traditionally been a manual process. Predictive analytics automates and optimizes underwriting by analyzing customer data, driving records, and historical claims. This enables insurers to tailor policies to individual needs, particularly for car insurance and auto insurance customers.
Identifying Fraudulent Claims Early On
Fraudulent claims cost insurers billions each year. By analyzing patterns in claim submissions, predictive analytics can flag anomalies, reducing the burden of fraud.
Benefits of Predictive Analytics for Insurance Companies
Cost Reduction and Increased Efficiency
Predictive analytics helps insurers streamline operations, reducing administrative costs and improving resource allocation. By predicting claim volumes, companies can prepare for peak times.
Personalized Customer Experience
By analyzing customer data, predictive analytics enables insurers to offer tailored solutions. For instance, personalized premium rates for auto insurance customers are determined by factors like driving habits and vehicle usage, enhancing customer loyalty.
Future of Predictive Analytics in the Insurance Industry
Emerging Trends and Technologies
As predictive analytics continue to evolve, insurers are adopting technologies like real-time data processing, IoT, and blockchain. These advancements help gather richer datasets, offering deeper insights into customer behavior and risk profiles.
Challenges and Considerations in Implementing Predictive Analytics
Data Privacy and Ethical Concerns
While predictive analytics offers numerous benefits, it raises concerns about data privacy and ethics. While these technologies add value to businesses, they also present challenges that insurers must navigate carefully:
Collection and Storage of Data: Insurers often collect sensitive information, such as health records, financial details, and location data, to build predictive models. Businesses must implement cybersecurity measures and comply with data protection regulations such as GDPR.
Transparency in Data Usage: Customers are often unaware of how their data is being collected, analyzed, or used in premium calculations. Insurers should clearly communicate their data practices, ensuring customers understand how predictive analytics benefits them.
Bias in Predictive Models: Predictive algorithms can unintentionally perpetuate biases if the training data reflect social inequalities. Insurers need to audit their models regularly to identify and mitigate bias.
Predictive analytics is revolutionizing the insurance industry. By enhancing risk assessment, reducing costs, and personalizing customer experiences, it empowers insurers to stay competitive in a rapidly changing market. As technology advances, the role of predictive analytics in the insurance sector is set to grow, paving the way for a smarter and more dynamic future.
The Role of Predictive Analytics in Insurance
The insurance industry has always relied on data to assess risks, set premiums, and manage claims. However, with the arrival of predictive analytics, insurers can now leverage advanced technologies to make more informed decisions. By analyzing historical data and predicting future outcomes, predictive analytics is transforming how companies operate, offering accuracy and efficiency.
What is Predictive Analytics and How Does It Work?
Predictive analytics involved using statistical techniques, machine learning algorithms, and data mining to forecast future events based on historical data. In the insurance industry, it enables companies to understand patterns and assess risks.
Key Technologies Behind Predictive Analytics in Insurance
Predictive analytics relies on several technologies:
Big Data: Insurers handle massive datasets that include everything from customer demographics and driving habits to external data sources such as weather reports and economic trends. Big data technologies enable the storage, processing, and real-time analysis of these datasets, making it possible to develop more accurate and comprehensive risk assessments.
ML Models: Machine learning algorithms form the core of predictive analytics. By training on historical data, machine learning models identify patterns and relationships that would be impossible for humans to detect manually. These models continuously improve as they are exposed to more data, allowing insurers to refine their predictions, whether for assessing risk or fraudulent claims.
Telematics and IoT Devices: These technologies are impactful in auto insurance. Devices installed in vehicles and or mobile apps track driving behavior, mileage, and even accident impact data. THe real-time information feeds directly into predictive models, allowing insurers to adjust premiums based on actual behavior.
Predictive Analytics Software: Specialized software solutions integrate the above technologies into a cohesive platform. These tools provide user-friendly interfaces for insurers to analyze data, generate predictive reports, and implement strategies.
How Predictive Analytics Improve Risk Assessment
Enhancing Underwriting with Data Driven Insights
Underwriting has traditionally been a manual process. Predictive analytics automates and optimizes underwriting by analyzing customer data, driving records, and historical claims. This enables insurers to tailor policies to individual needs, particularly for car insurance and auto insurance customers.
Identifying Fraudulent Claims Early On
Fraudulent claims cost insurers billions each year. By analyzing patterns in claim submissions, predictive analytics can flag anomalies, reducing the burden of fraud.
Benefits of Predictive Analytics for Insurance Companies
Cost Reduction and Increased Efficiency
Predictive analytics helps insurers streamline operations, reducing administrative costs and improving resource allocation. By predicting claim volumes, companies can prepare for peak times.
Personalized Customer Experience
By analyzing customer data, predictive analytics enables insurers to offer tailored solutions. For instance, personalized premium rates for auto insurance customers are determined by factors like driving habits and vehicle usage, enhancing customer loyalty.
Future of Predictive Analytics in the Insurance Industry
Emerging Trends and Technologies
As predictive analytics continue to evolve, insurers are adopting technologies like real-time data processing, IoT, and blockchain. These advancements help gather richer datasets, offering deeper insights into customer behavior and risk profiles.
Challenges and Considerations in Implementing Predictive Analytics
Data Privacy and Ethical Concerns
While predictive analytics offers numerous benefits, it raises concerns about data privacy and ethics. While these technologies add value to businesses, they also present challenges that insurers must navigate carefully:
Collection and Storage of Data: Insurers often collect sensitive information, such as health records, financial details, and location data, to build predictive models. Businesses must implement cybersecurity measures and comply with data protection regulations such as GDPR.
Transparency in Data Usage: Customers are often unaware of how their data is being collected, analyzed, or used in premium calculations. Insurers should clearly communicate their data practices, ensuring customers understand how predictive analytics benefits them.
Bias in Predictive Models: Predictive algorithms can unintentionally perpetuate biases if the training data reflect social inequalities. Insurers need to audit their models regularly to identify and mitigate bias.
Predictive analytics is revolutionizing the insurance industry. By enhancing risk assessment, reducing costs, and personalizing customer experiences, it empowers insurers to stay competitive in a rapidly changing market. As technology advances, the role of predictive analytics in the insurance sector is set to grow, paving the way for a smarter and more dynamic future.
The Role of Predictive Analytics in Insurance
The insurance industry has always relied on data to assess risks, set premiums, and manage claims. However, with the arrival of predictive analytics, insurers can now leverage advanced technologies to make more informed decisions. By analyzing historical data and predicting future outcomes, predictive analytics is transforming how companies operate, offering accuracy and efficiency.
What is Predictive Analytics and How Does It Work?
Predictive analytics involved using statistical techniques, machine learning algorithms, and data mining to forecast future events based on historical data. In the insurance industry, it enables companies to understand patterns and assess risks.
Key Technologies Behind Predictive Analytics in Insurance
Predictive analytics relies on several technologies:
Big Data: Insurers handle massive datasets that include everything from customer demographics and driving habits to external data sources such as weather reports and economic trends. Big data technologies enable the storage, processing, and real-time analysis of these datasets, making it possible to develop more accurate and comprehensive risk assessments.
ML Models: Machine learning algorithms form the core of predictive analytics. By training on historical data, machine learning models identify patterns and relationships that would be impossible for humans to detect manually. These models continuously improve as they are exposed to more data, allowing insurers to refine their predictions, whether for assessing risk or fraudulent claims.
Telematics and IoT Devices: These technologies are impactful in auto insurance. Devices installed in vehicles and or mobile apps track driving behavior, mileage, and even accident impact data. THe real-time information feeds directly into predictive models, allowing insurers to adjust premiums based on actual behavior.
Predictive Analytics Software: Specialized software solutions integrate the above technologies into a cohesive platform. These tools provide user-friendly interfaces for insurers to analyze data, generate predictive reports, and implement strategies.
How Predictive Analytics Improve Risk Assessment
Enhancing Underwriting with Data Driven Insights
Underwriting has traditionally been a manual process. Predictive analytics automates and optimizes underwriting by analyzing customer data, driving records, and historical claims. This enables insurers to tailor policies to individual needs, particularly for car insurance and auto insurance customers.
Identifying Fraudulent Claims Early On
Fraudulent claims cost insurers billions each year. By analyzing patterns in claim submissions, predictive analytics can flag anomalies, reducing the burden of fraud.
Benefits of Predictive Analytics for Insurance Companies
Cost Reduction and Increased Efficiency
Predictive analytics helps insurers streamline operations, reducing administrative costs and improving resource allocation. By predicting claim volumes, companies can prepare for peak times.
Personalized Customer Experience
By analyzing customer data, predictive analytics enables insurers to offer tailored solutions. For instance, personalized premium rates for auto insurance customers are determined by factors like driving habits and vehicle usage, enhancing customer loyalty.
Future of Predictive Analytics in the Insurance Industry
Emerging Trends and Technologies
As predictive analytics continue to evolve, insurers are adopting technologies like real-time data processing, IoT, and blockchain. These advancements help gather richer datasets, offering deeper insights into customer behavior and risk profiles.
Challenges and Considerations in Implementing Predictive Analytics
Data Privacy and Ethical Concerns
While predictive analytics offers numerous benefits, it raises concerns about data privacy and ethics. While these technologies add value to businesses, they also present challenges that insurers must navigate carefully:
Collection and Storage of Data: Insurers often collect sensitive information, such as health records, financial details, and location data, to build predictive models. Businesses must implement cybersecurity measures and comply with data protection regulations such as GDPR.
Transparency in Data Usage: Customers are often unaware of how their data is being collected, analyzed, or used in premium calculations. Insurers should clearly communicate their data practices, ensuring customers understand how predictive analytics benefits them.
Bias in Predictive Models: Predictive algorithms can unintentionally perpetuate biases if the training data reflect social inequalities. Insurers need to audit their models regularly to identify and mitigate bias.
Predictive analytics is revolutionizing the insurance industry. By enhancing risk assessment, reducing costs, and personalizing customer experiences, it empowers insurers to stay competitive in a rapidly changing market. As technology advances, the role of predictive analytics in the insurance sector is set to grow, paving the way for a smarter and more dynamic future.
FAQ
Is B2Metric compatible with my existing data systems?
Yes, B2Metric is designed to integrate smoothly with a wide range of data systems, ensuring a seamless fit within your insurance company’s current setup.
What are the steps to get started with B2Metric in my insurance company?
Is B2Metric compatible with my existing data systems?
Yes, B2Metric is designed to integrate smoothly with a wide range of data systems, ensuring a seamless fit within your insurance company’s current setup.
What are the steps to get started with B2Metric in my insurance company?
Is B2Metric compatible with my existing data systems?
Yes, B2Metric is designed to integrate smoothly with a wide range of data systems, ensuring a seamless fit within your insurance company’s current setup.
What are the steps to get started with B2Metric in my insurance company?
Is B2Metric compatible with my existing data systems?
Yes, B2Metric is designed to integrate smoothly with a wide range of data systems, ensuring a seamless fit within your insurance company’s current setup.
What are the steps to get started with B2Metric in my insurance company?
Related Blogs
Related Blogs
Related Blogs
Related Blogs
Dec 3, 2024
Hyper-Personalization in Banking: Meeting Customers Where They Are
Hyper-Personalization in Banking: Meeting Customers Where They Are
Nov 25, 2024
How Predictive Analytics is Revolutionizing the Insurance Industry?
How Predictive Analytics is Revolutionizing the Insurance Industry?
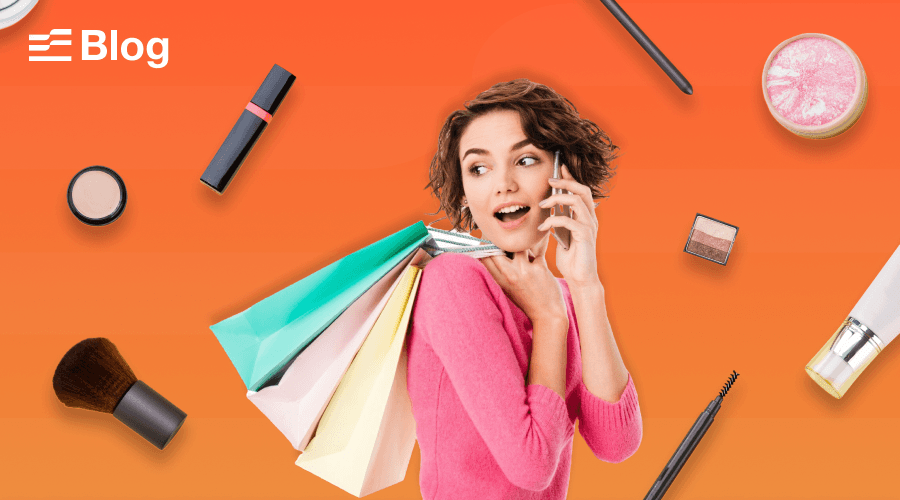
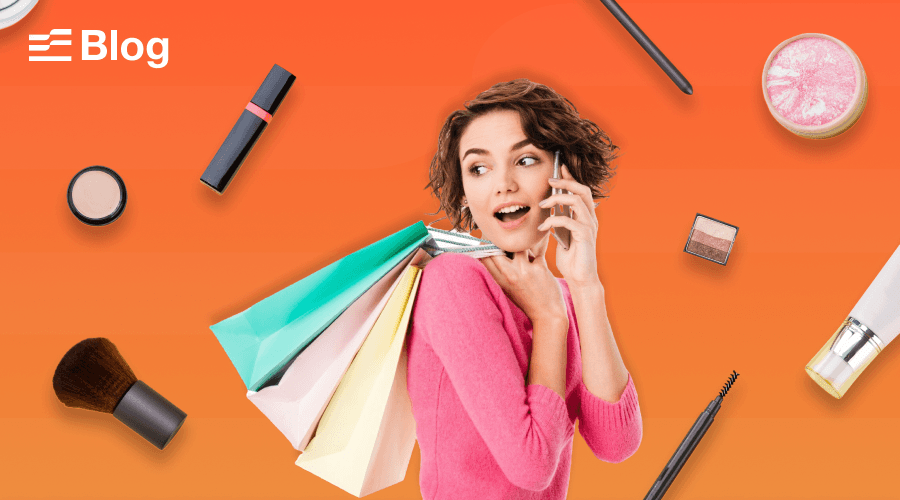
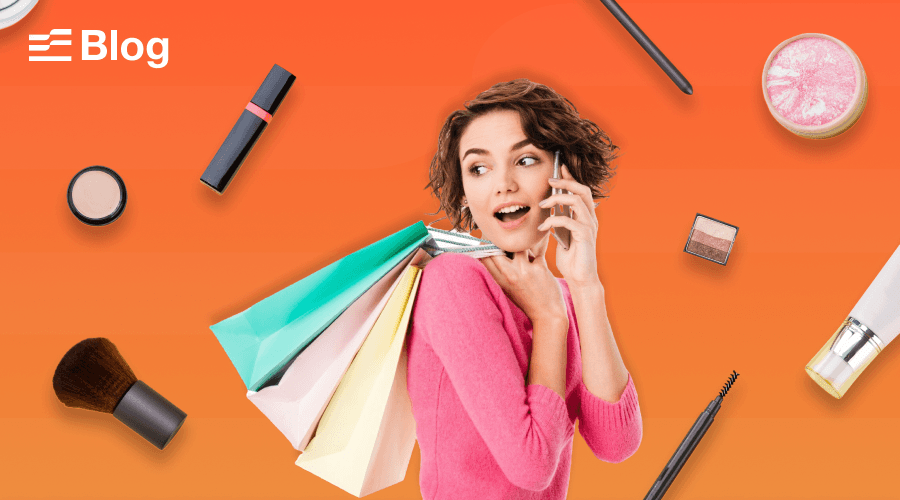
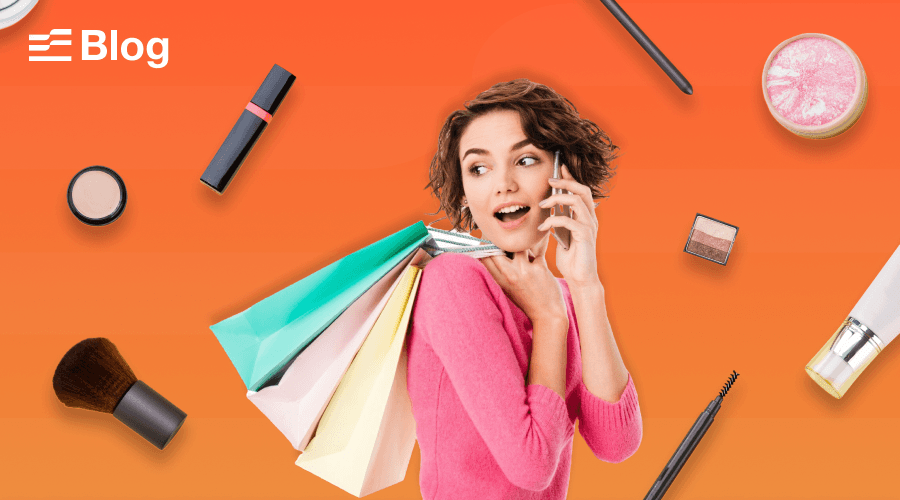
Nov 19, 2024
Maximize Customer Retention on Cyber Monday 2024: Proven Strategies
Maximize Customer Retention on Cyber Monday 2024: Proven Strategies
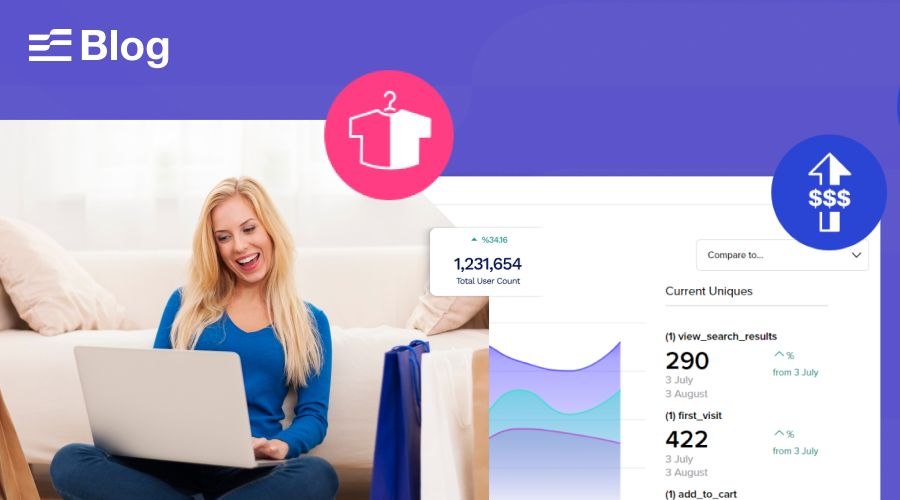
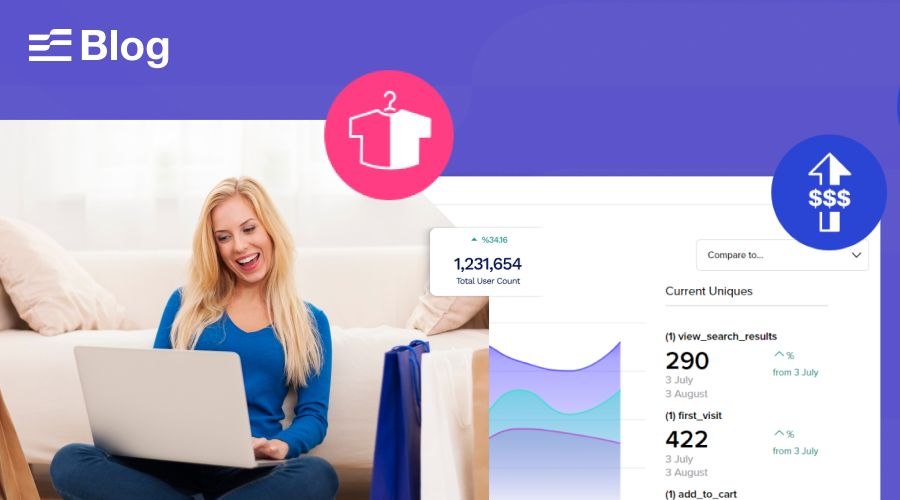
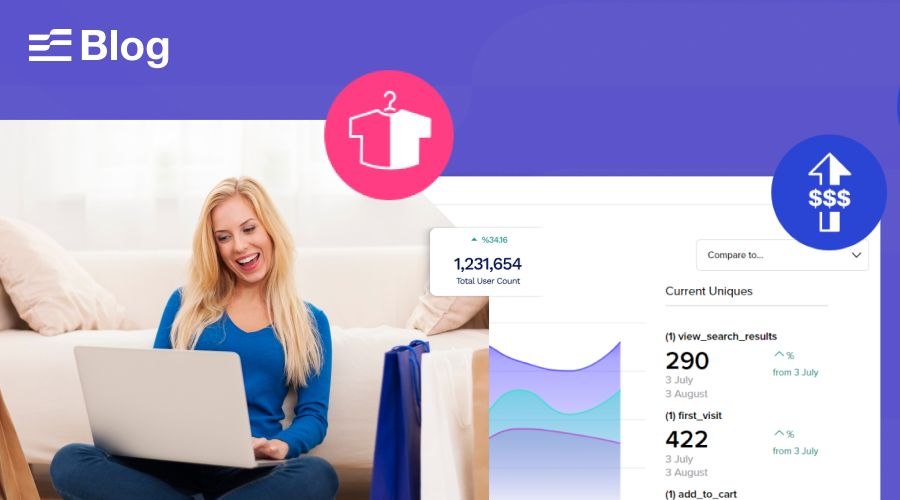
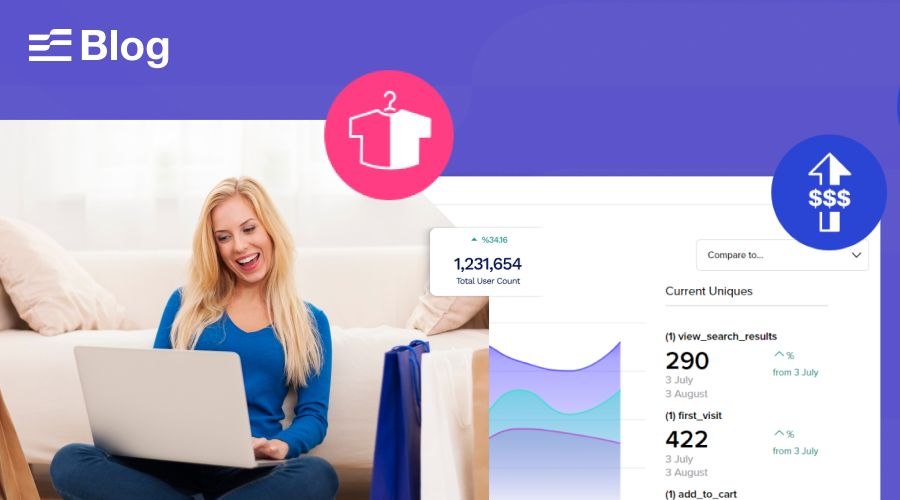
Nov 15, 2024
Predictive Analysis for Black Friday 2024: What You Need to Know
Predictive Analysis for Black Friday 2024: What You Need to Know
Product
Resources
Copyright © 2025 B2Metric | All Rights Reserved
Product
Resources
Copyright © 2025 B2Metric | All Rights Reserved
Customer intelligence data platform that helps brands analyze and predict user behavior across multi-channels.
Product
Top Blogs
Subscribe to our newsletter
Get the latest from B2Metric! 👀
Product
Subscribe to our newsletter!
Copyright © 2025 B2Metric | All Rights Reserved