
Hacer Yaldizli
Product Specialist
|
|
|
June 24, 2024
Jun 24, 2024
Jun 24, 2024
Jun 24, 2024
Technical Insights
Technical Insights
Technical Insights
7
7
7
7
min reading
min reading
min reading
min reading
Data
Data
Data
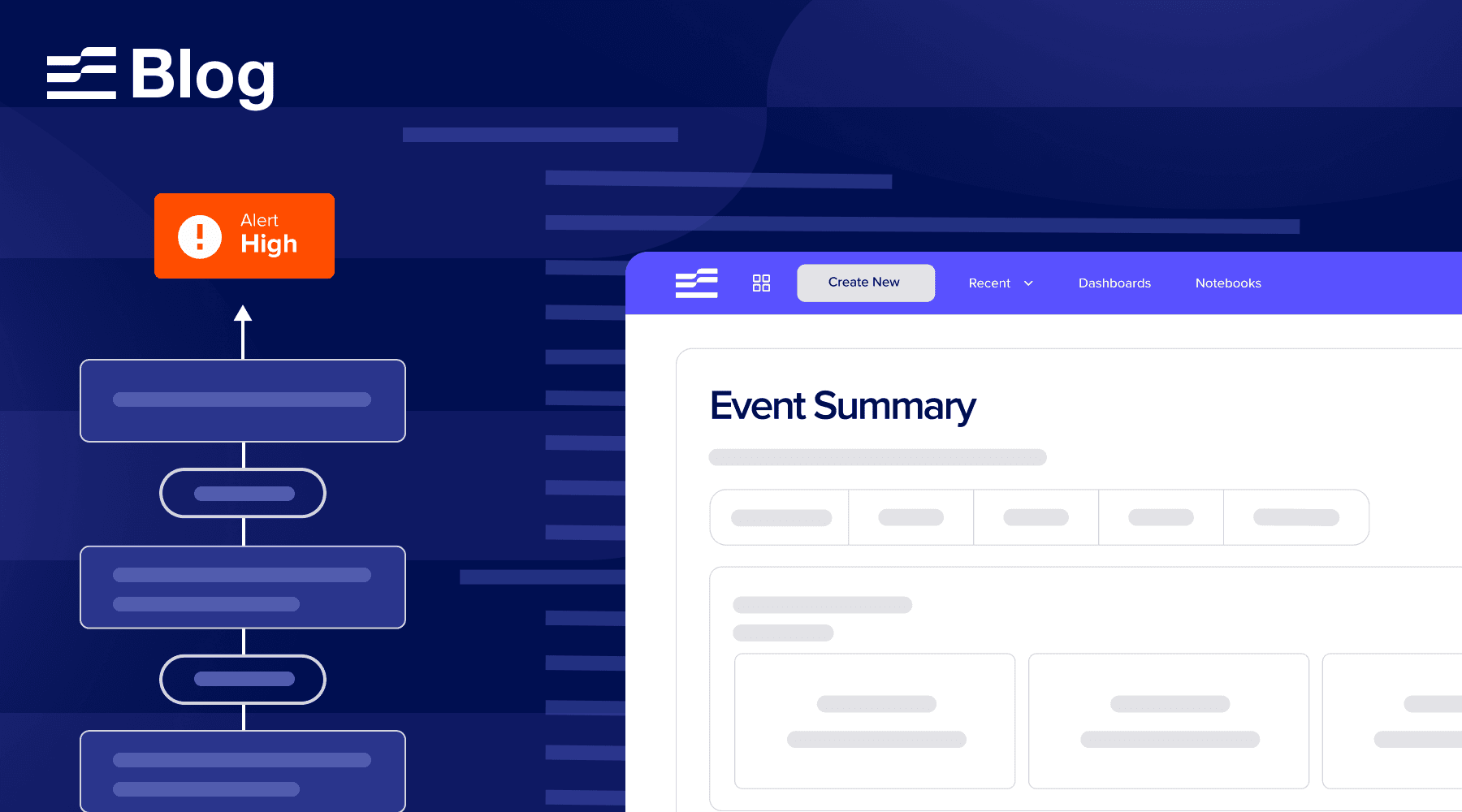
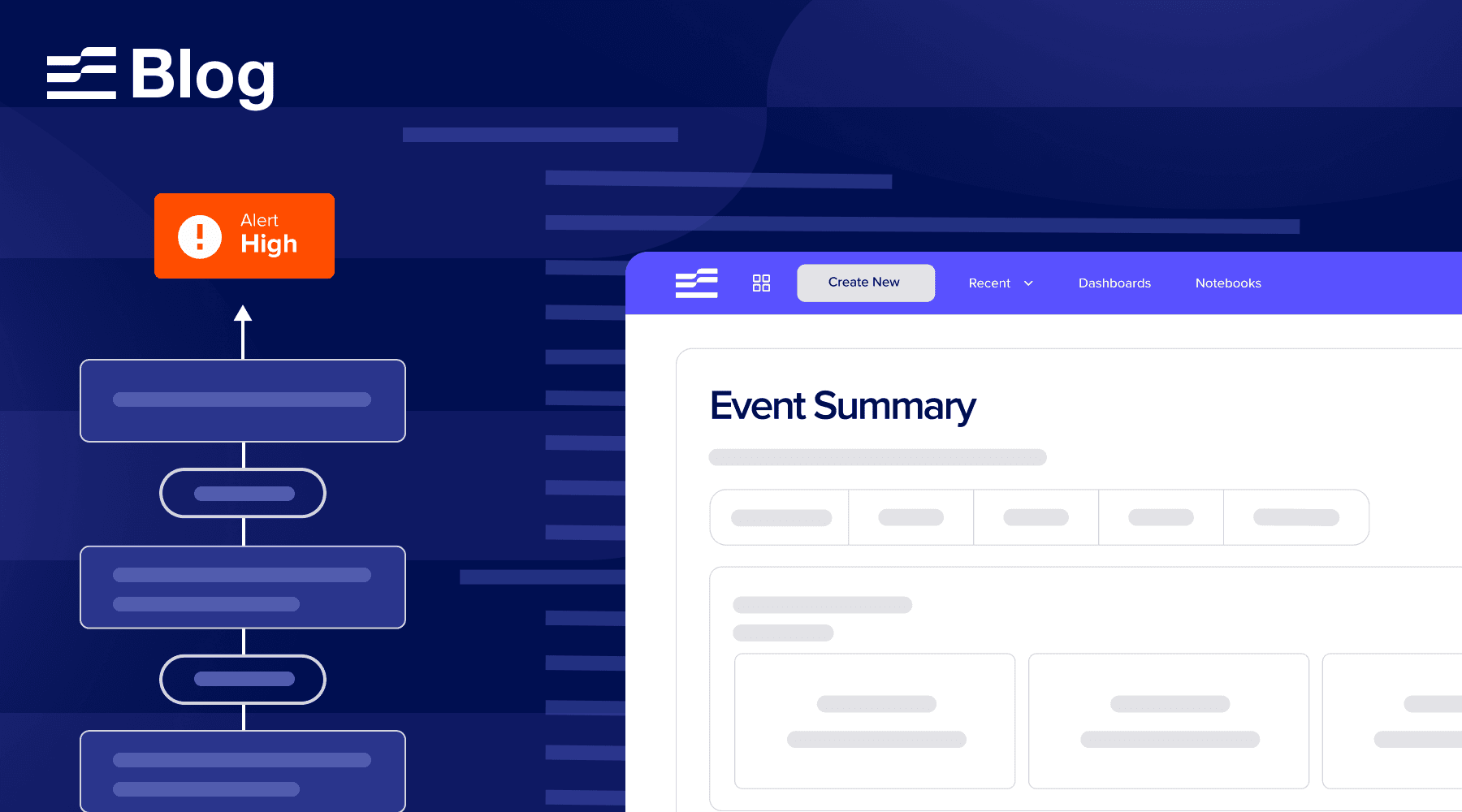
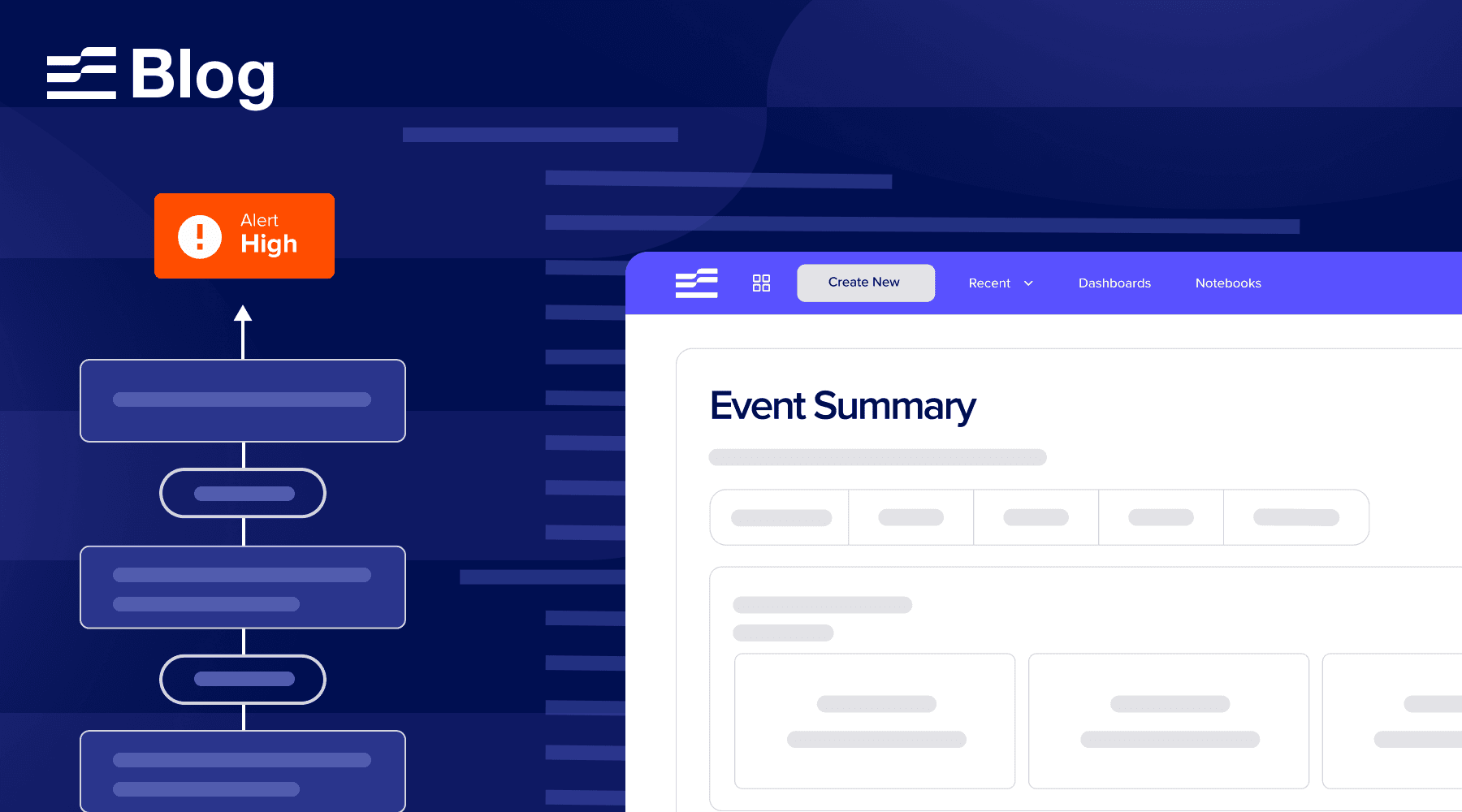
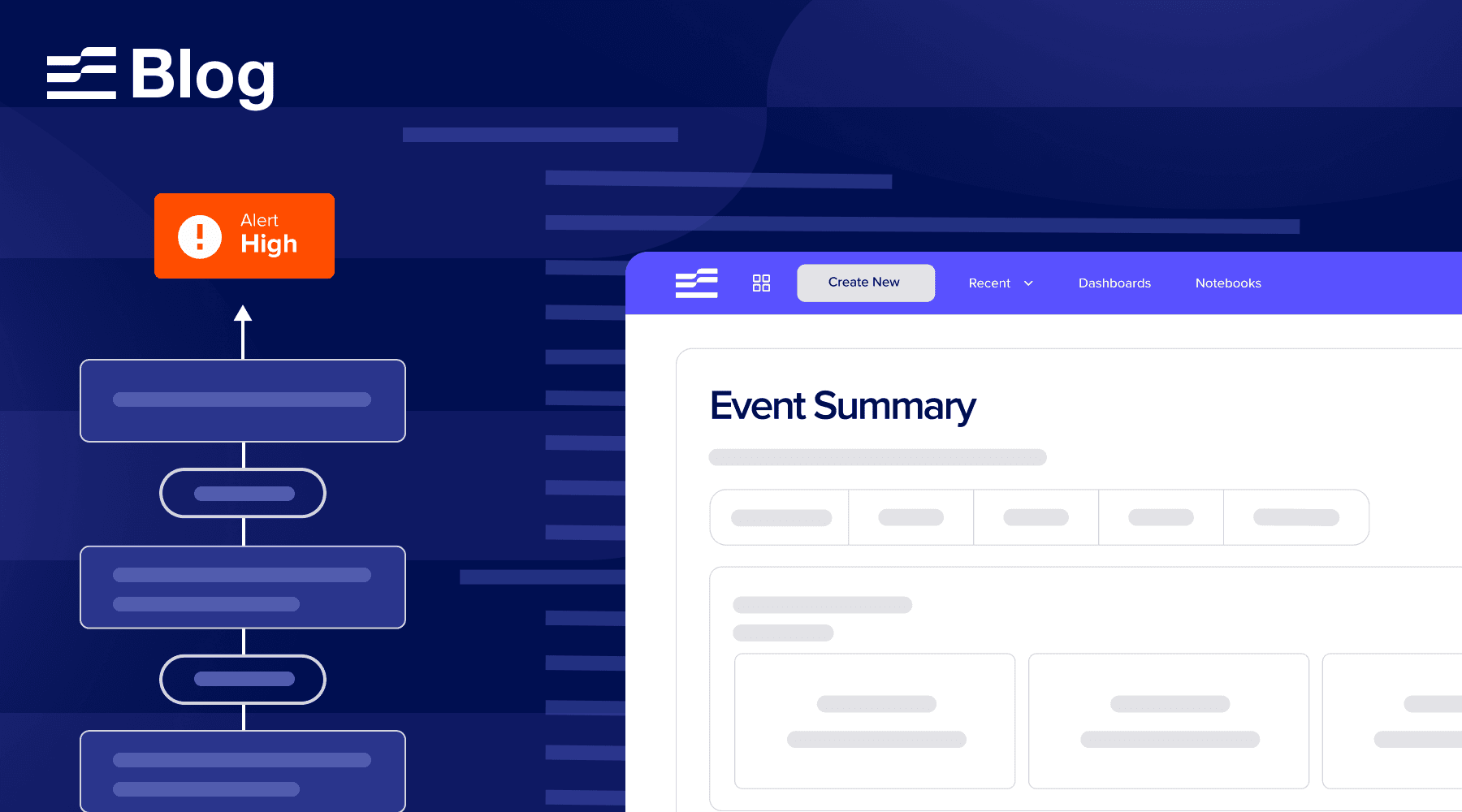
Table of contents
In the realm of data analysis and scientific research, the concepts of correlation and causation are fundamental. However, they are often misunderstood or used interchangeably, leading to incorrect conclusions. This guide aims to clarify the distinction between correlation and causation, their importance, and how to properly identify and use them in analysis.
Definition of correlation
Correlation explains how two factors, actions, or events are related. This relationship can be positive, where both factors increase together, or negative, where one decreases as the other decreases.
Positive Correlation: When one variable increases, the other variable also increases. For example, there is a positive correlation between the amount of time spent studying and exam scores.
Negative Correlation: When one variable increases, the other variable decreases. For instance, there is a negative correlation between the amount of time spent watching TV and physical fitness levels.
Zero Correlation: No relationship exists between the two variables. An example would be the correlation between shoe size and intelligence.
Definition of causation
Causation is when an event directly leads to a result. To establish causation, there must be a clear connection where the result wouldn't occur without the event. In contrast, correlation merely indicates a relationship between two factors without explaining why. For instance, while higher employee bonuses may correlate with increased sales revenue, this doesn't necessarily prove that bonuses cause higher sales—it could be coincidental or influenced by other factors like seasonal demand.
Why is it important to understand the distinction between correlation and causation?
Understanding the distinction between correlation and causation is crucial for making informed decisions and drawing accurate conclusions in various fields, including data analysis, research, and decision-making processes:
Avoiding Misinterpretations: Recognizing that correlation does not imply causation prevents misinterpreting relationships between variables. It reminds us not to assume a direct cause-and-effect relationship based solely on observed correlations.
Making Informed Decisions: Properly identifying causal relationships helps in making effective decisions. It allows us to focus resources on factors that truly influence outcomes, rather than on coincidental or indirect relationships.
Developing Effective Strategies: Understanding causation enables the development of strategies that target the root causes of problems or aim to enhance desired outcomes directly, leading to more effective interventions.
Minimizing Risks: Incorrectly assuming causation based on correlation can lead to costly mistakes or ineffective policies. By understanding causation, organizations can mitigate risks associated with flawed assumptions.
Enhancing Predictive Accuracy: Distinguishing between correlation and causation improves the accuracy of predictive models. It helps in forecasting outcomes more reliably by incorporating causal factors rather than relying solely on correlated variables.
Practical Example: Comprehensive Analysis of Ice Cream Sales and Drowning Incidents
1. Correlation:
Observation: During the summer months, there is a noticeable increase in both ice cream sales and drowning incidents.
Data: Statistical analysis reveals a positive correlation between the two variables; as ice cream sales rise, so do drowning incidents.
Explanation: The correlation between ice cream sales and drowning incidents does not imply a direct causal relationship between the two. Instead, it suggests that they tend to increase or decrease together over time.
2. Causation:
Confounding Variable: The likely cause behind this correlation is a third variable, known as a confounding variable, which influences both ice cream sales and drowning incidents.
Example: In this case, the confounding variable is hot weather. During the summer, hot weather prompts people to seek ways to cool down, leading to increased consumption of ice cream and more frequent visits to bodies of water for swimming and recreational activities.
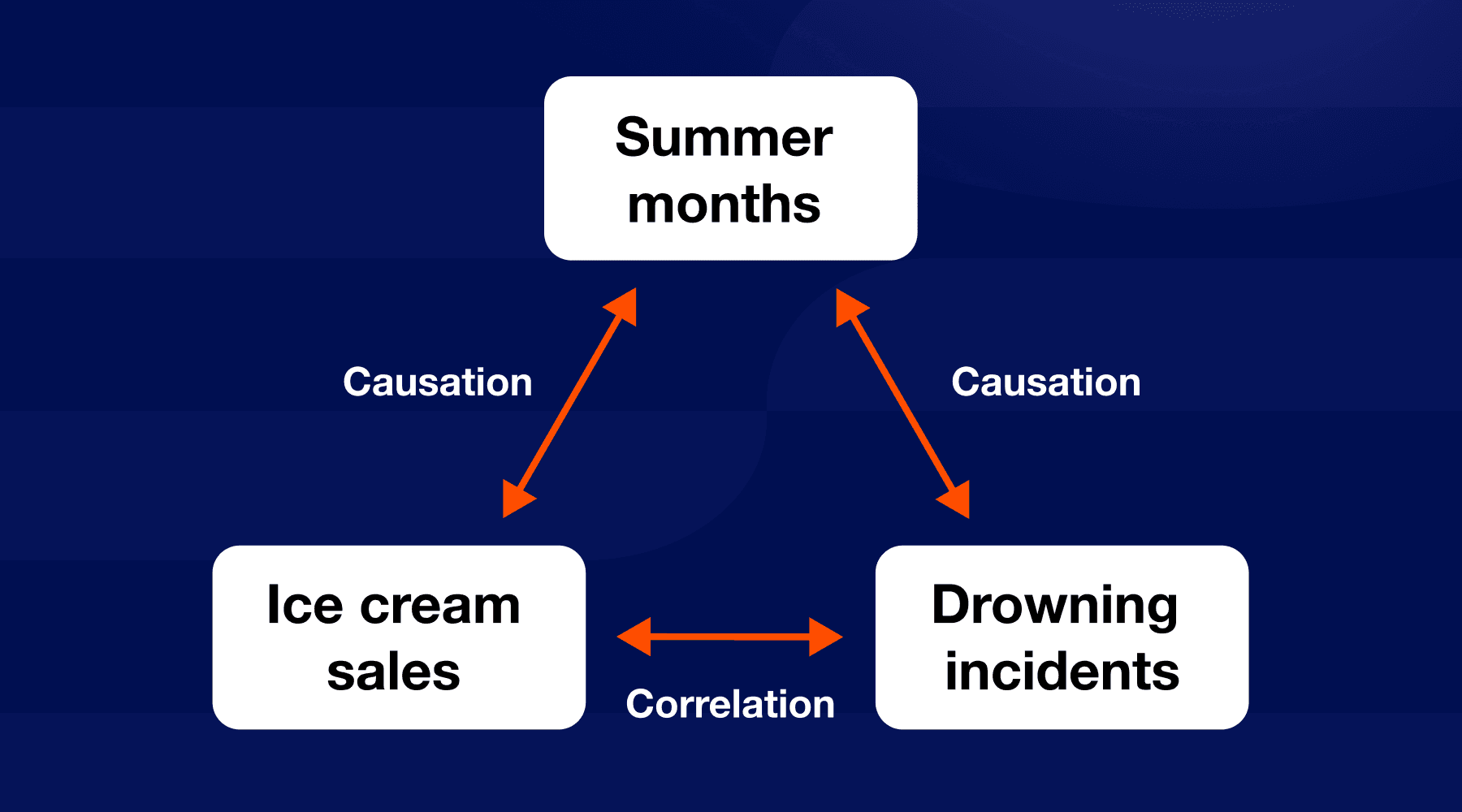
Mechanism: Hot weather increases the demand for ice cream as a refreshing treat, and it also encourages people to engage in water-related activities to cool off. Unfortunately, increased swimming activity also raises the risk of drowning incidents.
Conclusion: While there is a clear correlation between ice cream sales and drowning incidents, the causative link is indirect and mediated by hot weather as a confounding variable. This example underscores the importance of considering confounding factors in statistical analysis and research to avoid attributing causation where it does not exist.
Methods for Testing Causation
Testing causation often requires strict methods to establish a clear relationship between variables.
Randomized Controlled Trials (RCTs):
The gold standard for establishing causation.
Participants were randomly assigned to treatment or control groups.
Ensures observed effects are due to the intervention, not other factors.
Longitudinal Studies:
Follow subjects over time to observe changes in variables.
Provides insights into how one variable influences another.
Useful when conducting RCTs is impractical or unethical.
Natural Experiments:
Occur when external events create controlled-like conditions.
Allows for observation of causal effects in real-world settings.
Example: Comparing health outcomes before and after environmental policy changes.
Instrumental Variables:
Use external factors as instruments to strengthen causal inference.
Instruments affect the independent variable but not directly the dependent variable.
Example: Using geographic variations in policies to study healthcare access and health outcomes.
Causal Inference Methods:
Advanced statistical techniques to infer causality from observational data.
Includes Propensity Score Matching, Difference-in-Differences, and Regression Discontinuity Design.
Each method addresses specific challenges in establishing causal relationships.
Accurate establishment of causation is essential for informed decision-making and effective interventions, ensuring policies and strategies are based on reliable evidence of causal relationships rather than mere correlations.
In the realm of data analysis and scientific research, the concepts of correlation and causation are fundamental. However, they are often misunderstood or used interchangeably, leading to incorrect conclusions. This guide aims to clarify the distinction between correlation and causation, their importance, and how to properly identify and use them in analysis.
Definition of correlation
Correlation explains how two factors, actions, or events are related. This relationship can be positive, where both factors increase together, or negative, where one decreases as the other decreases.
Positive Correlation: When one variable increases, the other variable also increases. For example, there is a positive correlation between the amount of time spent studying and exam scores.
Negative Correlation: When one variable increases, the other variable decreases. For instance, there is a negative correlation between the amount of time spent watching TV and physical fitness levels.
Zero Correlation: No relationship exists between the two variables. An example would be the correlation between shoe size and intelligence.
Definition of causation
Causation is when an event directly leads to a result. To establish causation, there must be a clear connection where the result wouldn't occur without the event. In contrast, correlation merely indicates a relationship between two factors without explaining why. For instance, while higher employee bonuses may correlate with increased sales revenue, this doesn't necessarily prove that bonuses cause higher sales—it could be coincidental or influenced by other factors like seasonal demand.
Why is it important to understand the distinction between correlation and causation?
Understanding the distinction between correlation and causation is crucial for making informed decisions and drawing accurate conclusions in various fields, including data analysis, research, and decision-making processes:
Avoiding Misinterpretations: Recognizing that correlation does not imply causation prevents misinterpreting relationships between variables. It reminds us not to assume a direct cause-and-effect relationship based solely on observed correlations.
Making Informed Decisions: Properly identifying causal relationships helps in making effective decisions. It allows us to focus resources on factors that truly influence outcomes, rather than on coincidental or indirect relationships.
Developing Effective Strategies: Understanding causation enables the development of strategies that target the root causes of problems or aim to enhance desired outcomes directly, leading to more effective interventions.
Minimizing Risks: Incorrectly assuming causation based on correlation can lead to costly mistakes or ineffective policies. By understanding causation, organizations can mitigate risks associated with flawed assumptions.
Enhancing Predictive Accuracy: Distinguishing between correlation and causation improves the accuracy of predictive models. It helps in forecasting outcomes more reliably by incorporating causal factors rather than relying solely on correlated variables.
Practical Example: Comprehensive Analysis of Ice Cream Sales and Drowning Incidents
1. Correlation:
Observation: During the summer months, there is a noticeable increase in both ice cream sales and drowning incidents.
Data: Statistical analysis reveals a positive correlation between the two variables; as ice cream sales rise, so do drowning incidents.
Explanation: The correlation between ice cream sales and drowning incidents does not imply a direct causal relationship between the two. Instead, it suggests that they tend to increase or decrease together over time.
2. Causation:
Confounding Variable: The likely cause behind this correlation is a third variable, known as a confounding variable, which influences both ice cream sales and drowning incidents.
Example: In this case, the confounding variable is hot weather. During the summer, hot weather prompts people to seek ways to cool down, leading to increased consumption of ice cream and more frequent visits to bodies of water for swimming and recreational activities.
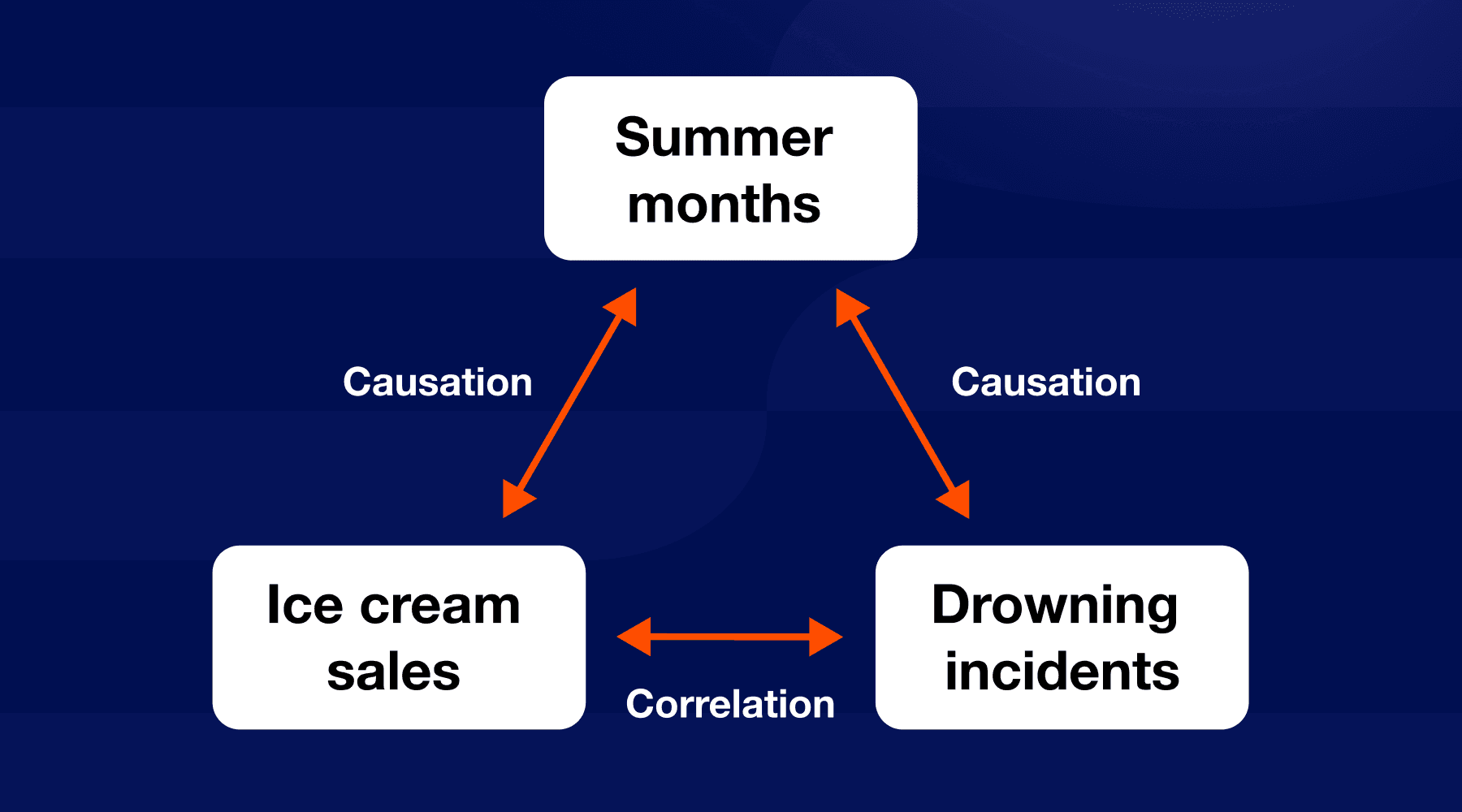
Mechanism: Hot weather increases the demand for ice cream as a refreshing treat, and it also encourages people to engage in water-related activities to cool off. Unfortunately, increased swimming activity also raises the risk of drowning incidents.
Conclusion: While there is a clear correlation between ice cream sales and drowning incidents, the causative link is indirect and mediated by hot weather as a confounding variable. This example underscores the importance of considering confounding factors in statistical analysis and research to avoid attributing causation where it does not exist.
Methods for Testing Causation
Testing causation often requires strict methods to establish a clear relationship between variables.
Randomized Controlled Trials (RCTs):
The gold standard for establishing causation.
Participants were randomly assigned to treatment or control groups.
Ensures observed effects are due to the intervention, not other factors.
Longitudinal Studies:
Follow subjects over time to observe changes in variables.
Provides insights into how one variable influences another.
Useful when conducting RCTs is impractical or unethical.
Natural Experiments:
Occur when external events create controlled-like conditions.
Allows for observation of causal effects in real-world settings.
Example: Comparing health outcomes before and after environmental policy changes.
Instrumental Variables:
Use external factors as instruments to strengthen causal inference.
Instruments affect the independent variable but not directly the dependent variable.
Example: Using geographic variations in policies to study healthcare access and health outcomes.
Causal Inference Methods:
Advanced statistical techniques to infer causality from observational data.
Includes Propensity Score Matching, Difference-in-Differences, and Regression Discontinuity Design.
Each method addresses specific challenges in establishing causal relationships.
Accurate establishment of causation is essential for informed decision-making and effective interventions, ensuring policies and strategies are based on reliable evidence of causal relationships rather than mere correlations.
In the realm of data analysis and scientific research, the concepts of correlation and causation are fundamental. However, they are often misunderstood or used interchangeably, leading to incorrect conclusions. This guide aims to clarify the distinction between correlation and causation, their importance, and how to properly identify and use them in analysis.
Definition of correlation
Correlation explains how two factors, actions, or events are related. This relationship can be positive, where both factors increase together, or negative, where one decreases as the other decreases.
Positive Correlation: When one variable increases, the other variable also increases. For example, there is a positive correlation between the amount of time spent studying and exam scores.
Negative Correlation: When one variable increases, the other variable decreases. For instance, there is a negative correlation between the amount of time spent watching TV and physical fitness levels.
Zero Correlation: No relationship exists between the two variables. An example would be the correlation between shoe size and intelligence.
Definition of causation
Causation is when an event directly leads to a result. To establish causation, there must be a clear connection where the result wouldn't occur without the event. In contrast, correlation merely indicates a relationship between two factors without explaining why. For instance, while higher employee bonuses may correlate with increased sales revenue, this doesn't necessarily prove that bonuses cause higher sales—it could be coincidental or influenced by other factors like seasonal demand.
Why is it important to understand the distinction between correlation and causation?
Understanding the distinction between correlation and causation is crucial for making informed decisions and drawing accurate conclusions in various fields, including data analysis, research, and decision-making processes:
Avoiding Misinterpretations: Recognizing that correlation does not imply causation prevents misinterpreting relationships between variables. It reminds us not to assume a direct cause-and-effect relationship based solely on observed correlations.
Making Informed Decisions: Properly identifying causal relationships helps in making effective decisions. It allows us to focus resources on factors that truly influence outcomes, rather than on coincidental or indirect relationships.
Developing Effective Strategies: Understanding causation enables the development of strategies that target the root causes of problems or aim to enhance desired outcomes directly, leading to more effective interventions.
Minimizing Risks: Incorrectly assuming causation based on correlation can lead to costly mistakes or ineffective policies. By understanding causation, organizations can mitigate risks associated with flawed assumptions.
Enhancing Predictive Accuracy: Distinguishing between correlation and causation improves the accuracy of predictive models. It helps in forecasting outcomes more reliably by incorporating causal factors rather than relying solely on correlated variables.
Practical Example: Comprehensive Analysis of Ice Cream Sales and Drowning Incidents
1. Correlation:
Observation: During the summer months, there is a noticeable increase in both ice cream sales and drowning incidents.
Data: Statistical analysis reveals a positive correlation between the two variables; as ice cream sales rise, so do drowning incidents.
Explanation: The correlation between ice cream sales and drowning incidents does not imply a direct causal relationship between the two. Instead, it suggests that they tend to increase or decrease together over time.
2. Causation:
Confounding Variable: The likely cause behind this correlation is a third variable, known as a confounding variable, which influences both ice cream sales and drowning incidents.
Example: In this case, the confounding variable is hot weather. During the summer, hot weather prompts people to seek ways to cool down, leading to increased consumption of ice cream and more frequent visits to bodies of water for swimming and recreational activities.
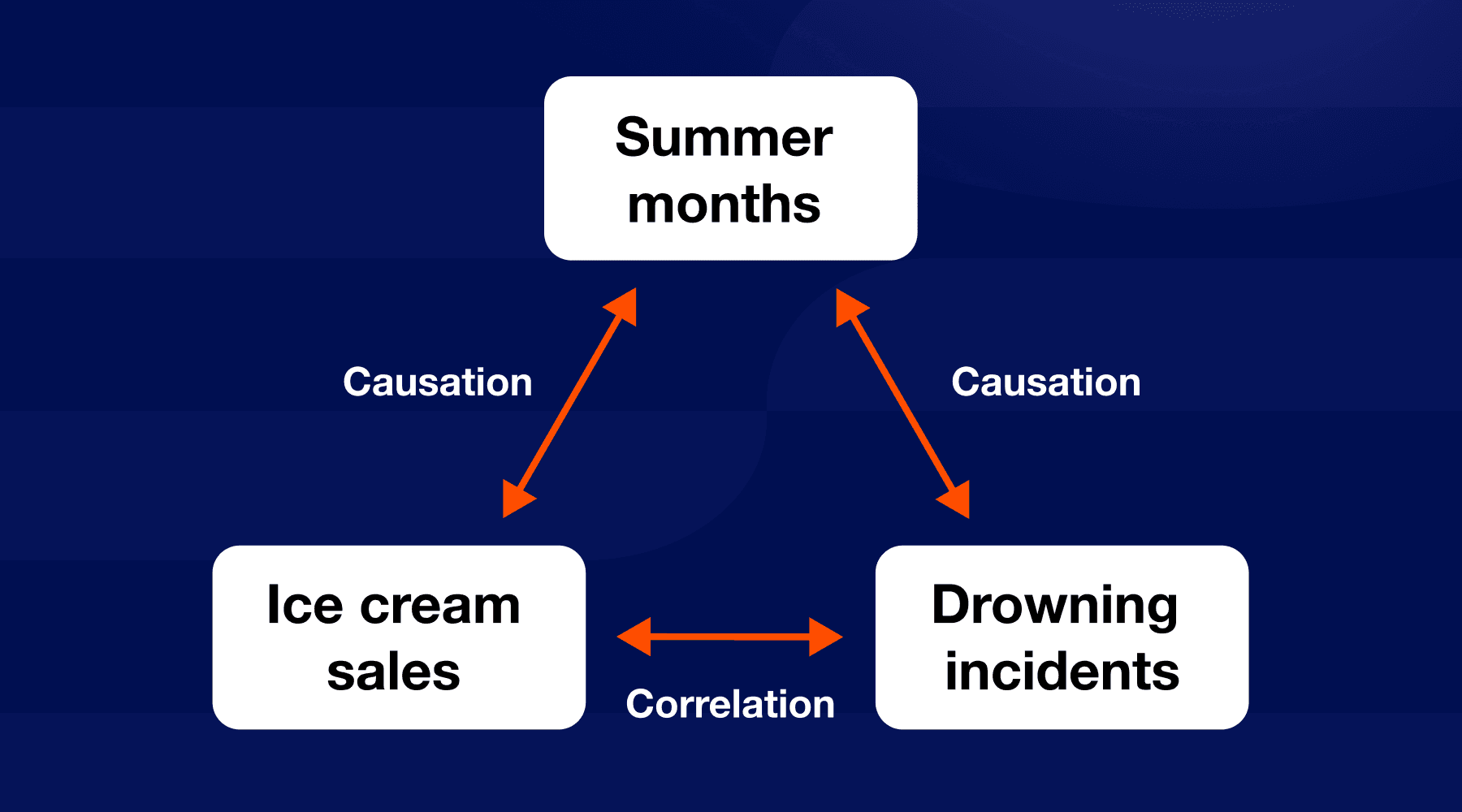
Mechanism: Hot weather increases the demand for ice cream as a refreshing treat, and it also encourages people to engage in water-related activities to cool off. Unfortunately, increased swimming activity also raises the risk of drowning incidents.
Conclusion: While there is a clear correlation between ice cream sales and drowning incidents, the causative link is indirect and mediated by hot weather as a confounding variable. This example underscores the importance of considering confounding factors in statistical analysis and research to avoid attributing causation where it does not exist.
Methods for Testing Causation
Testing causation often requires strict methods to establish a clear relationship between variables.
Randomized Controlled Trials (RCTs):
The gold standard for establishing causation.
Participants were randomly assigned to treatment or control groups.
Ensures observed effects are due to the intervention, not other factors.
Longitudinal Studies:
Follow subjects over time to observe changes in variables.
Provides insights into how one variable influences another.
Useful when conducting RCTs is impractical or unethical.
Natural Experiments:
Occur when external events create controlled-like conditions.
Allows for observation of causal effects in real-world settings.
Example: Comparing health outcomes before and after environmental policy changes.
Instrumental Variables:
Use external factors as instruments to strengthen causal inference.
Instruments affect the independent variable but not directly the dependent variable.
Example: Using geographic variations in policies to study healthcare access and health outcomes.
Causal Inference Methods:
Advanced statistical techniques to infer causality from observational data.
Includes Propensity Score Matching, Difference-in-Differences, and Regression Discontinuity Design.
Each method addresses specific challenges in establishing causal relationships.
Accurate establishment of causation is essential for informed decision-making and effective interventions, ensuring policies and strategies are based on reliable evidence of causal relationships rather than mere correlations.
Related articles
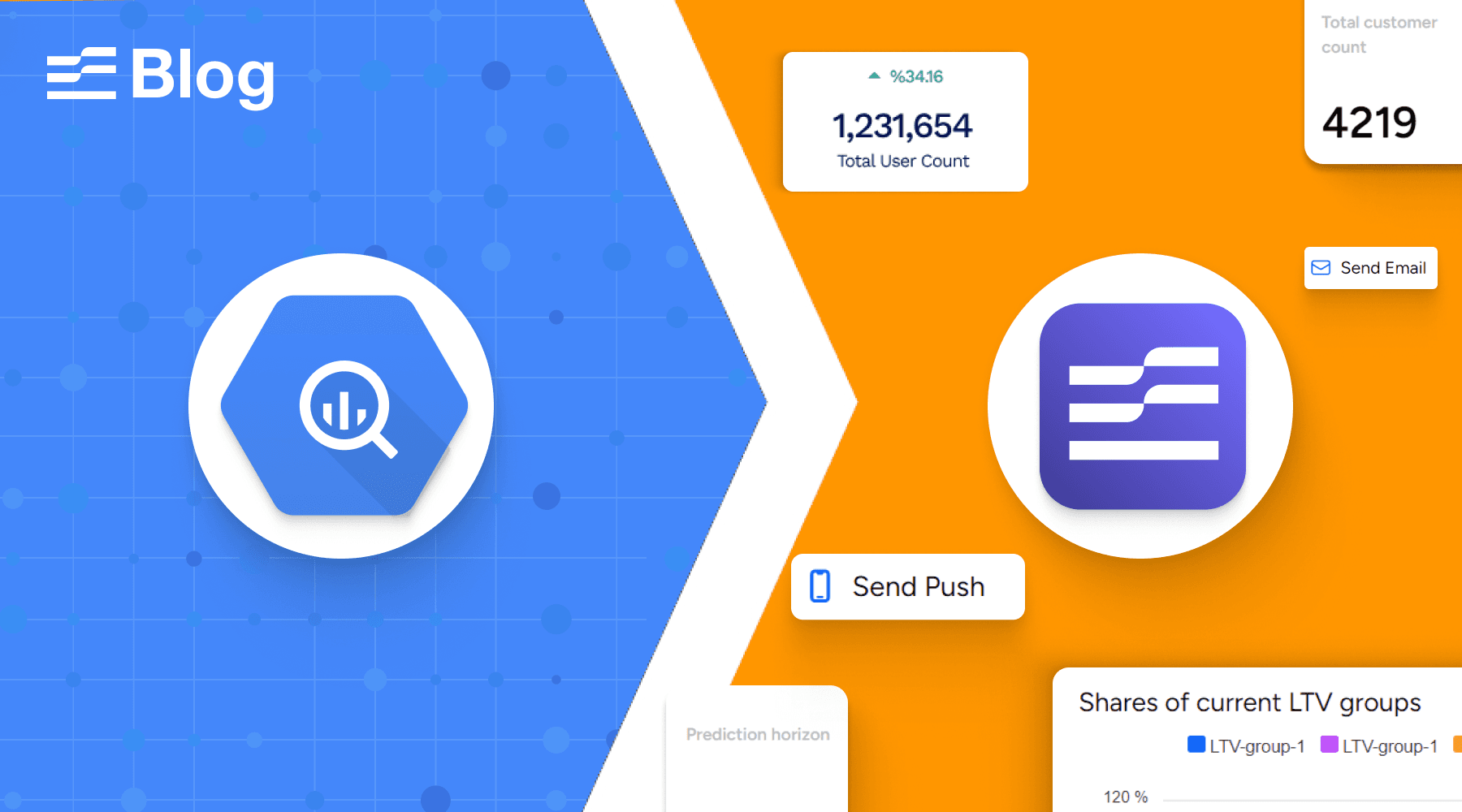
Jun 14, 2024
|
Data
Why Do I Need B2metric When I Have Bigquery?
BigQuery as a key element of your data framework is a smart strategic move. BigQuery embodies effectiveness and user friendliness qualities that resonate with our team at B2metric.

Jul 2, 2024
|
Marketing
What is Engagement Rate? How to Calculate Engagement Rate?
What is Engagement Rate? How to Calculate Engagement Rate?
FAQ
How Does B2Metric’s AI-Based CDP Work?
What Are the Advantages of Using an AI-Based CDP?
How Can B2Metric’s AI-Based CDP Help You Understand Customer Behavior?
How Does B2Metric’s AI-Based CDP Work?
What Are the Advantages of Using an AI-Based CDP?
How Can B2Metric’s AI-Based CDP Help You Understand Customer Behavior?
How Does B2Metric’s AI-Based CDP Work?
What Are the Advantages of Using an AI-Based CDP?
How Can B2Metric’s AI-Based CDP Help You Understand Customer Behavior?
How Does B2Metric’s AI-Based CDP Work?
What Are the Advantages of Using an AI-Based CDP?
How Can B2Metric’s AI-Based CDP Help You Understand Customer Behavior?
Related Blogs
Related Blogs
Related Blogs
Related Blogs
Dec 3, 2024
Hyper-Personalization in Banking: Meeting Customers Where They Are
Hyper-Personalization in Banking: Meeting Customers Where They Are
Nov 25, 2024
How Predictive Analytics is Revolutionizing the Insurance Industry?
How Predictive Analytics is Revolutionizing the Insurance Industry?
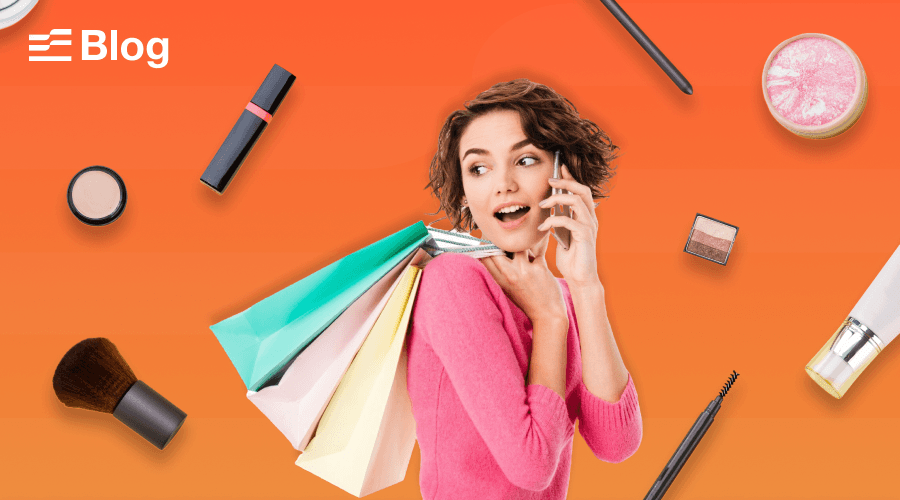
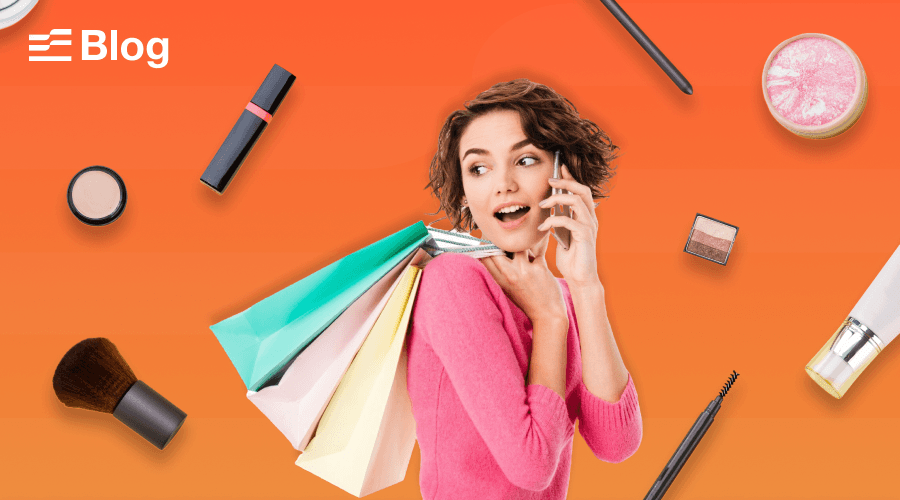
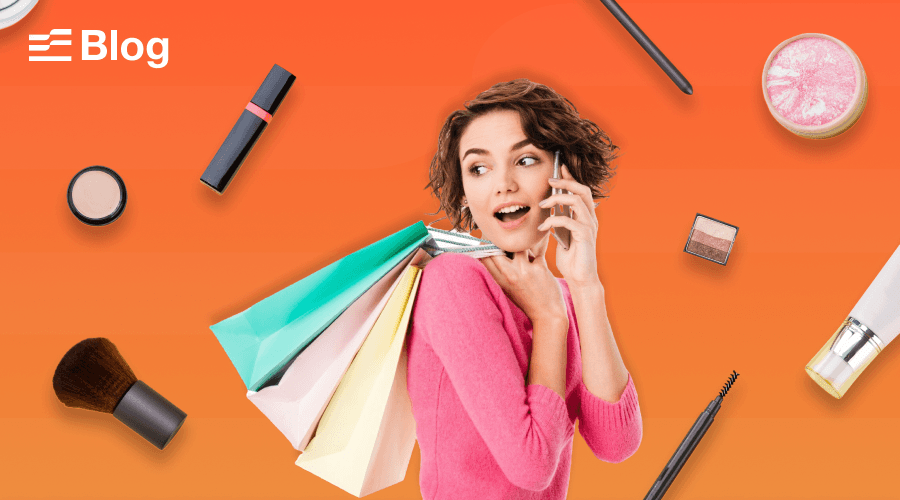
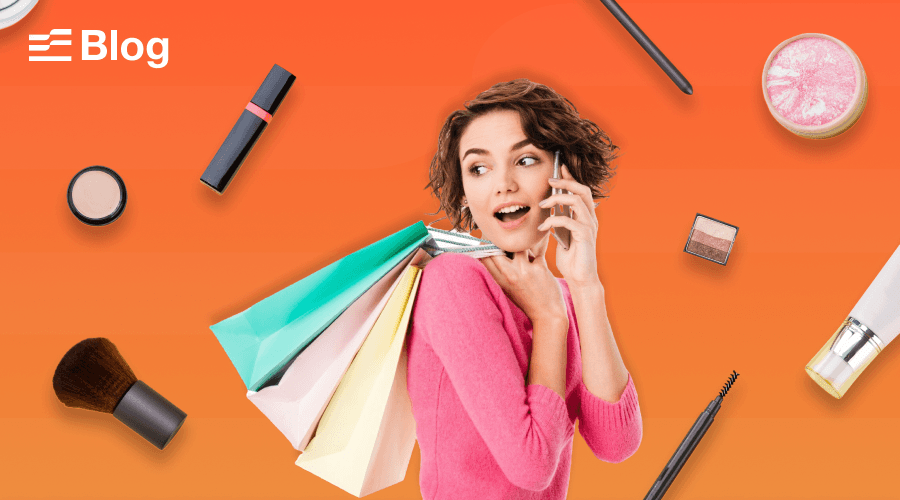
Nov 19, 2024
Maximize Customer Retention on Cyber Monday 2024: Proven Strategies
Maximize Customer Retention on Cyber Monday 2024: Proven Strategies
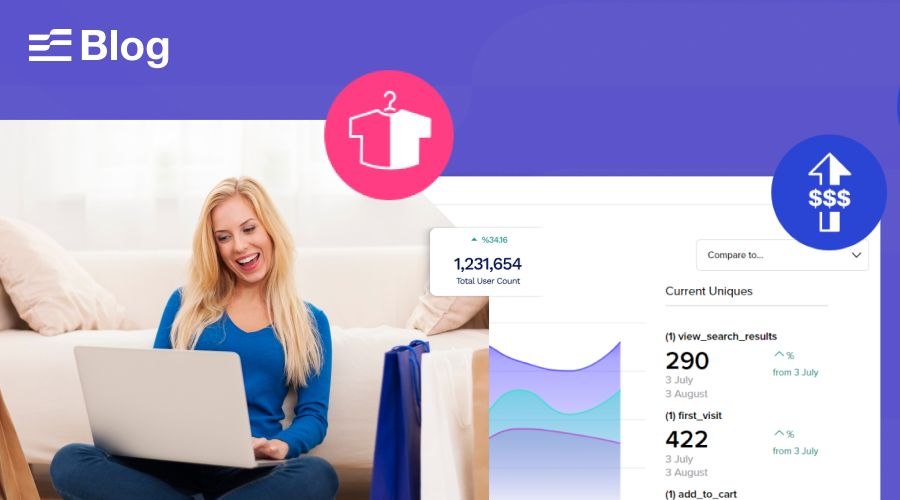
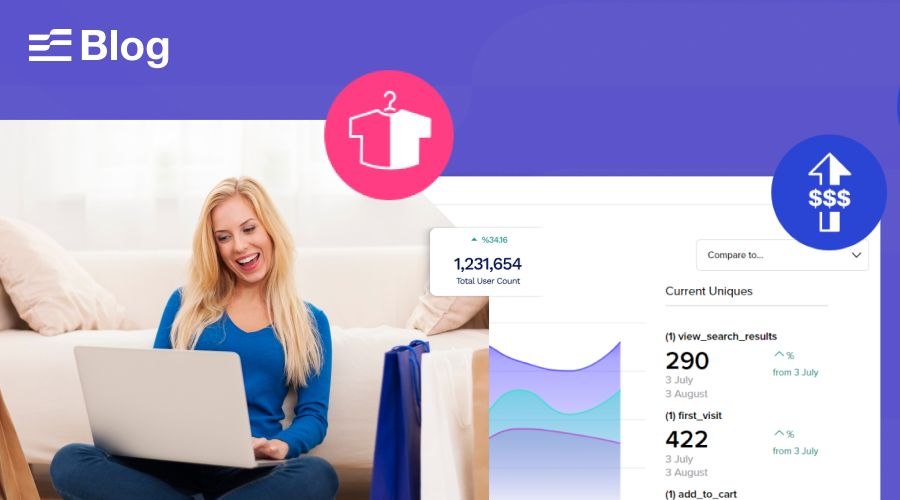
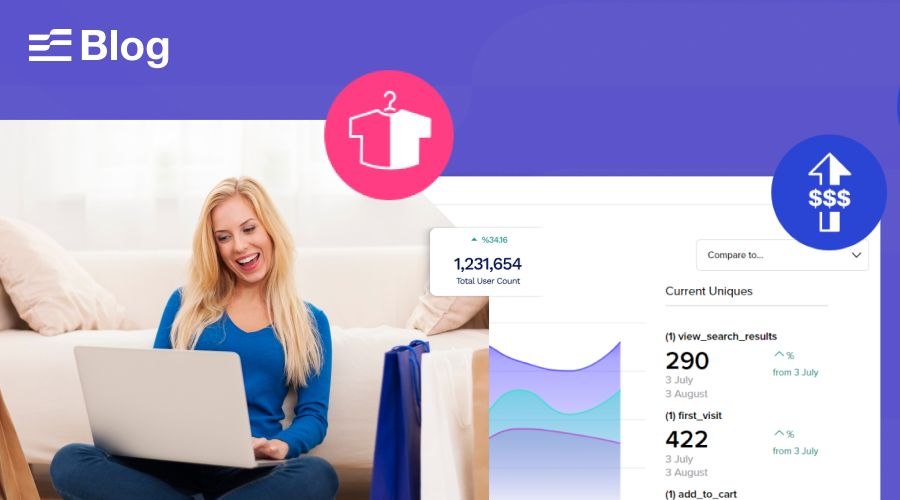
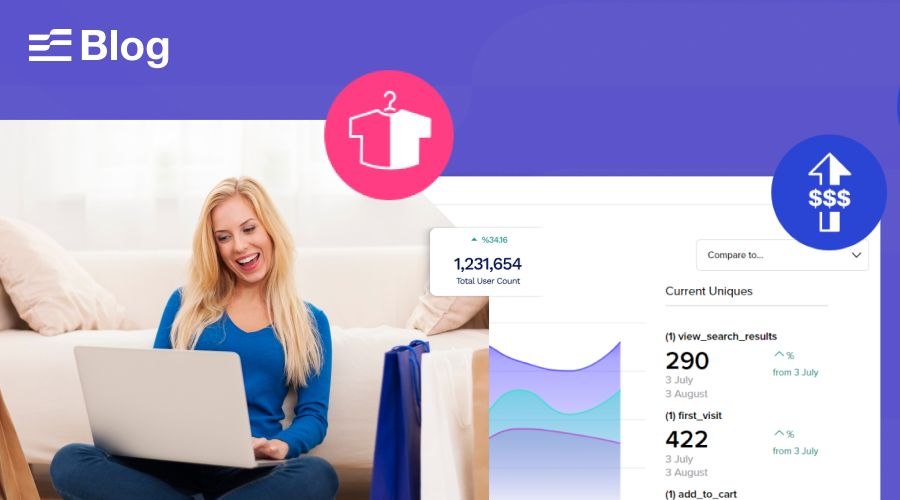
Nov 15, 2024
Predictive Analysis for Black Friday 2024: What You Need to Know
Predictive Analysis for Black Friday 2024: What You Need to Know
Customer intelligence data platform that helps brands analyze and predict user behavior across multi-channels.
Product
Resources
Top Blogs
Subscribe to our newsletter
Get the latest from B2Metric! 👀
Customer intelligence data platform that helps brands analyze and predict user behavior across multi-channels.
Product
Subscribe to our newsletter
Get the latest from B2Metric! 👀
Subscribe to our newsletter
Lorem ipsum dolor sit amet consectetur adipiscing elit aliquam mauris sed ma
Customer intelligence data platform that helps brands analyze and predict user behavior across multi-channels.
Product
Top Blogs
Subscribe to our newsletter
Get the latest from B2Metric! 👀
Customer intelligence data platform that helps brands analyze and predict user behavior across multi-channels.
Product
Top Blogs
Subscribe to our newsletter
Get the latest from B2Metric! 👀